Difference between revisions of "Design Criteria of Methods"
m |
|||
Line 19: | Line 19: | ||
== What are scientific methods? == | == What are scientific methods? == | ||
− | Generally speaking, scientific methods create knowledge. This knowledge creation process follows certain principles and has a certain rigour. Knowledge that is created through scientific methods should be ideally [https://plato.stanford.edu/entries/scientific-reproducibility/ ''reproducible''], which means that someone else under the given circumstances would come up with the same insights when using the same respective methods. This is insofar important, as other people would maybe create different data under a similar setting, but all the data should answer research questions or hypotheses in the same way. However, there are some methods that may create different knowledge patterns, which is why documentation is pivotal in the application of scientific methods. Some forms of knowledge, such as the perception of individuals, cannot be reproduced, because these are singular perspectives. '''Knowledge created through scientific methods hence either follows a systematic application of methods, or a systematic documentation of the application of methods'''. Reproducible approaches create the same | + | Generally speaking, scientific methods create knowledge. This knowledge creation process follows certain principles and has a certain rigour. Knowledge that is created through scientific methods should be ideally [https://plato.stanford.edu/entries/scientific-reproducibility/ ''reproducible''], which means that someone else under the given circumstances would come up with the same insights when using the same respective methods. This is insofar important, as other people would maybe create different data under a similar setting, but all the data should answer research questions or hypotheses in the same way. However, there are some methods that may create different knowledge patterns, which is why documentation is pivotal in the application of scientific methods. Some forms of knowledge, such as the perception of individuals, cannot be reproduced, because these are singular perspectives. '''Knowledge created through scientific methods hence either follows a systematic application of methods, or a systematic documentation of the application of methods'''. Reproducible approaches ideally create the same patterns, and other approaches should be equally well documented to safeguard that for all steps taken it can be understood what was being done precisely. |
Another possibility to define methods concerns the different stages of research in which they are applied. '''Methods are about gathering data, analysing data, and interpreting data.''' Not all methods do all of these three steps, in fact most methods are even exclusive to one or two of these steps. For instance, one may analyse [[Semi-structured Interview|structured interviews]] - which are one way to gather data - with statistical tests, which are a form of analysis. The interpretation of the results is then built around the design of the interviews, and there are norms and much experience concerning the interpretation of statistical tests. Established methods often follow certain more or less established norms, and the norms can be broken down into the main ''design criteria of methods''. Let us have a look at these. | Another possibility to define methods concerns the different stages of research in which they are applied. '''Methods are about gathering data, analysing data, and interpreting data.''' Not all methods do all of these three steps, in fact most methods are even exclusive to one or two of these steps. For instance, one may analyse [[Semi-structured Interview|structured interviews]] - which are one way to gather data - with statistical tests, which are a form of analysis. The interpretation of the results is then built around the design of the interviews, and there are norms and much experience concerning the interpretation of statistical tests. Established methods often follow certain more or less established norms, and the norms can be broken down into the main ''design criteria of methods''. Let us have a look at these. | ||
Line 45: | Line 45: | ||
* Temporal scale: [[:Category:Past|Past]] - [[:Category:Present|Present]] - [[:Category:Future|Future]] | * Temporal scale: [[:Category:Past|Past]] - [[:Category:Present|Present]] - [[:Category:Future|Future]] | ||
− | Try to understand how methods can be operationalised in view of these criteria. Some methods only tick one option per criterion, and others may span across many different combinations of criteria. Out of the [[Methods|great diversity of methods]], this categorization gives you a first positioning of each specific method. There are literally hundreds of methods out there. Instead of understanding all of them, try to understand the criteria that unite and differentiate them. Only through an understanding of these methodological design criteria may you be able to choose the method that creates the knowledge that may be needed. | + | Try to understand how methods can be operationalised in view of these criteria. Some methods only tick one option per criterion, and others may span across many different combinations of criteria. Out of the [[Methods|great diversity of methods]], this categorization gives you a first positioning of each specific method. There are literally hundreds of methods out there. Instead of understanding all of them, try to understand the criteria that unite and differentiate them. Only through an understanding of these methodological design criteria may you be able to choose the method that creates the knowledge that may be needed. |
= Additional Information = | = Additional Information = |
Revision as of 11:21, 20 October 2023
Note: The German version of this entry can be found here: Design Criteria of Methods (German)
Disclaimer: The entry on the history of scientific methods outlines in detail how we came to the current situation, and explains why our thinking about scientific methods - and with it our thinking about science in general - will shift due to and compared to the past. This article on design criteria, in turn, focuses more on the present, and even more importantly, the future.
In short: This entry provides a critical overview and understanding of the methodological canon of science. It presents the underlying conceptualisation of scientific methods that guides the categorisation of the presented methods on this Wiki.
Contents
Why change the perspective on methods?
A strong reasoning why we need a different way to think about scientific methods is actually rooted in the history of science, and how the way we think about methods in the future should be different from these past developments. Kuhn talks about revolution and paradigm shifts in scientific thinking, and many would firmly argue that such a paradigm shift is immanent right now. Actually, it is already happening.
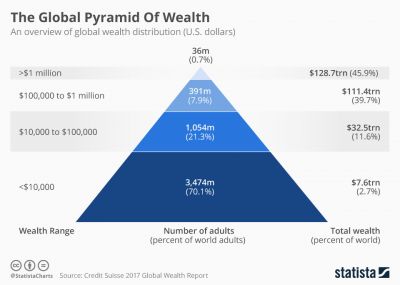
Today, we face unprecedented challenges in terms of magnitude and complexity. While some problems are still easy to solve, others are not. Due to the complex phenomena of globalisation we now face challenges that are truly spanning across the globe, and may as well affect the whole globe. Globalisation once started with the promise of a creation of a united and connected humankind. This is of course a very naive worldview and, as we see today, has failed in many regards. Instead, we see a rise in global inequality in terms of many indicators, among them global wealth distribution. Climate change, biodiversity loss and ocean acidification showcase that the challenges are severe, and may as well lead to an end of the world as we know it, if you are a pessimist. If you are not a pessimist, then stewing in a grim scenario of our future demise will not help us to prevent this from happening. Instead, sustainability science and many other branches of science opted for a solution orientated agenda, which proclaims that instead of merely describing problems, and despite the strong urge to complain about it, we need to work together and find solutions for these wicked problems. Over the last few decades, science inched its way slowly out of its ivory towers, and the necessity of science and society working together emerged. While this will often bring us closer to potential solutions that ideally serve more and more people, science is still stuck in a mode that is often arrogant, resource driven and anachronistic. While this is surely a harsh word, some people call this "normal science". However, the current challenges demand a new mode of science; one that is radical yet rooted in long traditions, collaborative yet able to focus deeply on specific parts of knowledge, solution orientated yet not naive, and peaceful instead of confrontational and resource-focussed.
Science has long realised that due to globalisation, shared and joined perspectives between disciplines and the benefits of modern communication, we - scientists from all disciplinary and cultural backgrounds - often have a shared interest in specific topics. For every topic, there are different scientific disciplines that focus on it, albeit from different perspectives. Take climate change, where many disciplines offer their five cent to the debate. Will any of the singular scientific disciplines solve the problems alone? Most likely not. Werewolves may be killed by silver bullets, yet for most problems we currently face, there is no silver bullet. We will have to think of more complex solutions, often working in unisono across different disciplines, and together with society.
However, most disciplines have established specific traditions when it comes to the methods that are being used. While these traditions guarantee experience, they may not allow us to solve the problem we currently face. Some methods are exclusive for certain disciplines, yet most methods are used by several disciplines. Sometimes these methods co-evolved, but more often than not the methods departed from a joined starting point and then become more specific in the respective context of the particular branch of science. For instance, interviews are widely used in many areas of science, yet the implementation is often very diverse, and specific branches of sciences typically favour specific types of interviews. This gets us stuck if we want to share insights, work together and combine knowledge gathered in different disciplines. Undisputedly, modern science increasingly builds on a canon of diverse approaches, and there is a clear necessity to unpack the ‘silos’ that methods are currently packed in. While nothing can be said against deep methodological expertise, some disciplines have the tendency to rely on their methods, and declare their approaches as the most valid ones. However, we all look at the Universe from different perspectives, through different lenses, and create different models. The choice of the scientific method strongly determines the outcome of our research. If someone insists on a specific method, then this person will receive the specific knowledge that this method is able to unlock. However, other parts of knowledge may be impossible to be unlocked through this method, and there is not a single method that can unlock all knowledge.
We need to choose and apply methods depending on the type of knowledge we aim to create, regardless of the disciplinary background or tradition. We should aim to become more and more experienced and empowered to use the method that is most ideal for each research purpose and not rely solely on what our discipline has always been doing. In order to achieve this, design criteria of methods can help to create a conceptualization of the nature of methods. In other words: what are the underlying principles that guide the available scientific methods? First, we need to start with the most fundamental question:
What are scientific methods?
Generally speaking, scientific methods create knowledge. This knowledge creation process follows certain principles and has a certain rigour. Knowledge that is created through scientific methods should be ideally reproducible, which means that someone else under the given circumstances would come up with the same insights when using the same respective methods. This is insofar important, as other people would maybe create different data under a similar setting, but all the data should answer research questions or hypotheses in the same way. However, there are some methods that may create different knowledge patterns, which is why documentation is pivotal in the application of scientific methods. Some forms of knowledge, such as the perception of individuals, cannot be reproduced, because these are singular perspectives. Knowledge created through scientific methods hence either follows a systematic application of methods, or a systematic documentation of the application of methods. Reproducible approaches ideally create the same patterns, and other approaches should be equally well documented to safeguard that for all steps taken it can be understood what was being done precisely.
Another possibility to define methods concerns the different stages of research in which they are applied. Methods are about gathering data, analysing data, and interpreting data. Not all methods do all of these three steps, in fact most methods are even exclusive to one or two of these steps. For instance, one may analyse structured interviews - which are one way to gather data - with statistical tests, which are a form of analysis. The interpretation of the results is then built around the design of the interviews, and there are norms and much experience concerning the interpretation of statistical tests. Established methods often follow certain more or less established norms, and the norms can be broken down into the main design criteria of methods. Let us have a look at these.
Quantitative vs Qualitative
One of the strongest discourses regarding the classification of methods revolves around the question whether a method is quantitative or qualitative. Quantitative methods focus on the measurement, counting and constructed generalisation, linking the statistical or mathematical analysis of data, as well as the interpretation of data that consists of numbers to extract patterns or support theories. Simply spoken, quantitative methods are about numbers. Qualitative methods, on the other hand, focus on the human dimensions of the observable or conceptual reality, often linking observational data or interpretation of existing data directly to theory or concepts, allowing for deep contextual understanding. Both quantitative and qualitative methods are normative. These two generally different lines of thinking are increasingly linked in recent decades, yet the majority of research - and more importantly, disciplines - are dominated by either qualitative or quantitative methods. While this is perfectly fine per se, there is a deep ideological trench between these two approaches, and much judgement is passed on which approach is more valid. This can be misleading if not wrong, and I propose instead to choose the appropriate approach depending on the intended knowledge. However, there is one caveat: much of the scientific canon of the last decades was dominated by research building on quantitative approaches. However, new exciting methods emerge especially in qualitative research. Since novel solutions are necessary for the problems we currently face, it seems necessary that the amount as well as the proportion of qualitative research increases in the future.
Deductive vs Inductive
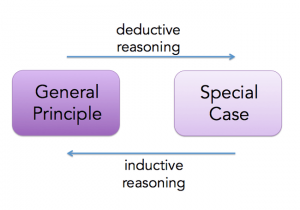
Methods can further be distinguished into whether they are inductive or deductive. This distinction is rooted deeply in the tradition of Francis Bacon. While Bacon can be clearly seen as being more leaning towards the inductive, he paved the road towards a clearer distinction between the inductive and the deductive. If there is such a thing as the scientific method at all, we owe much of the systematic behind it to Bacon.
Deductive reasoning builds on statements or theories that are confirmed by observation or can be confirmed by logic. It has become an important baseline of especially the natural sciences and research utilising quantitative methods Inductive methods draw conclusions based on data or observations. Many argue that these inferences are only approximations of a probable truth. Deriving the knowledge from data and not from theory in inductive research can be seen as a counterpoint to hypothesis-driven deductive reasoning, although this is not always the case. While research even today is mostly stated to be clearly either inductive or deductive, this is often a hoax. You have many folks using large datasets and stating clear hypotheses. If these folks were honest, it would become clear that they analysed the data until they had these hypotheses, yet they publish the results with hypotheses they pretend they had from the very beginning. This is a clear indicator of scientific tradition getting back at us which, up until today, is often built on a culture that demands hypothesis building. Then again, other research is equally dogmatic in demanding an inductive approach, widely rejecting any deduction. We will not discuss the merits of inductive reasoning here, but instead only highlight that its openness and urge to avoid being deterministic provides an important and relevant counterpoint to deductive research. Just as in the realms of quantitative and qualitative methods, there is a deep trench between inductive and deductive research. One possibility to overcome this in an honest and valuable approach would be abductive reasoning, which consists of iterations between data and theory. Modern research is actually framed and conducted in such a way with a constant exchange between data and theory. Considering the complexity of modern research, this also makes sense: on the one hand, not all research can be broken down into small "hypothesisable" units, and on the other hand, so much knowledge already exists, which is why much research builds on previous theories and knowledge. Still, today, and for years to come, we will have to acknowledge that much of modern research will fall into the traditionally established inductive or deductive category. It is therefore not surprising that critical theory and other approaches cast doubt over these rigid criteria, and the knowledge they produce. Nevertheless, they represent an important categorisation for the current state of the art of many areas of science, and therefore deserve critical attention, at least because many methods imply either one or the other approach.
Spatial and Temporal Scales
Different scientific methods are focussing on or even restricted to certain spatial scales, yet others may span across scales. Some methods are operationalised on a global scale, while others focus on the individual. For instance, a statistical correlation analysis can be applied on data about individuals gathered in a survey, but also be applied to global economic data. A global spatial scale of sampling is defined by data that covers the whole globe, or a non-deliberately chosen part of the globe. Such global analyses are of increasing importance, as they allow us to have a truly united understanding of societal and natural phenomena that concern the global scale. An individual scale of sampling is a scale that focuses on individual objects, which includes people and other living entities. The individual scale can give us deep insight into living beings, with a pronounced focus on research on humans. Since our concepts of humans are diverse, there are also many diverse approaches found here, and many branches of science focus on the individual. This scale certainly gained importance over the last decades, and we are only starting to understand the diversity of knowledge that an individual scale has to offer.
In between, there is a huge void that can have different names in different domains of science - ‘landscapes’, ‘systems’, ‘institutions’, ‘catchments’, and others. Different branches of science focus on different mid-scales, yet this is often considered to be one of the most abundantly investigated scales, since it can generate a great diversity of data. We will call it the ‘system scale’. A system scale of sampling is defined by any scale that contains several individual objects that interact or are embedded in a wider matrix surrounding. Regarding our understanding of systems, this is a relevant methodological scale, because it can generate a great diversity of empirical data, and many diverse methods are applied at this scale. In addition, it is also quite a relevant scale in terms of normativity because it can generate knowledge about change in systems which can be operationalised with the goal to foster or hinder such change. Hence, this system scale can be quite relevant in terms of knowledge that focuses on policy. Changing the globe takes certainly longer, and understanding change in people has many deeper theoretical foundations and frameworks.
The last criterion to conceptualise methods concerns time. The vast majority of empirical studies look at one slice of time (the present), and this makes the studies that try to understand the past or make the bold attempt to understand more about the future all the more precious. Analysis of the past can be greatly diverse, from quantitative longitudinal data analysis to deep hermeneutical text analysis and interpretation of writers long gone. There are many windows into the past, and we only start to unravel many of the most fascinating perspectives, as much of the treasures available are only now investigated by science. Still, there is a long tradition in history studies, cultural studies, and many more branches of sciences that prove how much we can learn from the past. With the utilisation of predictive models, the development of scenarios about the future and many other available approaches, science also attempts to generate more knowledge about what might become. The very concept of the future is a human privilege that science increasingly focuses on because of the many challenges we face. New approaches emerged over the last decades, and our knowledge about the future certainly grows. To date, we have to acknowledge that these studies are still all too rare, and much of our empirical knowledge builds on snapshots of a certain point in time. If you want to learn more about the design criterion of time, please refer to the entry on Time.
Based on these considerations, one needs to remember the following criteria when you approach a concrete scientific method:
- Quantitative - Qualitative
- Inductive - Deductive
- Spatial scale: Individual - System - Global
- Temporal scale: Past - Present - Future
Try to understand how methods can be operationalised in view of these criteria. Some methods only tick one option per criterion, and others may span across many different combinations of criteria. Out of the great diversity of methods, this categorization gives you a first positioning of each specific method. There are literally hundreds of methods out there. Instead of understanding all of them, try to understand the criteria that unite and differentiate them. Only through an understanding of these methodological design criteria may you be able to choose the method that creates the knowledge that may be needed.
Additional Information
Von Wehrden, H., Luederitz, C., Leventon, J., & Russell, S. (2017). Methodological Challenges in Sustainability Science: a call for method plurality, procedural rigor and longitudinal research. Challenges in Sustainability, 5(1). https://doi.org/10.12924/cis2017.05010035
The author of this entry is Henrik von Wehrden.