System Boundaries
In short: Based on the idea of System Thinking, this entry discusses how to properly draw boundaries in systems.
System thinking has emerged during the last decades as one of the most important approaches in sustainability science. Originating in the initial system theory, several conceptual frameworks and data analysis methodologies have been developed that aim to generate a better understanding of the interactive dynamics within a typically spatially bound system. While much attention has been hence drawn to a better theory development and subsequent application within a defined setting, less attention has been aimed at a definition of system boundaries.
The problem with System Boundaries
While boundaries of some isolated systems as well as simple theoretical models can be rather clearly defined, much attention of the recent literature has actually focussed on the effects that systemic interactions create across distances, which have historically been considered separate see for example teleconnections in atmospheric research. It is now evident that inland watersheds are linked to oceangraphic processes through freshwater and nutrient inputs, although these have historically and disciplinarily been examined separately. Furthermore, the nestedness of systems is another challenge - the idea that smaller systems are contained within, and are the constituent components of, larger systems such as organs within the larger integrated functionality of the human body. Some organs can be removed or replaced, but only in relation to the overall functions they provide and how they fit or match the context of the overall body (i.e, blood type or immune response). Government jurisdictions give other clear examples, where countries may contain several provinces as part of a federal system, which in turn can contain smaller administrative units, creating a multi-level administrative whole.
The debate often resonates around a void between the global and the local levels of social and ecological scales such as space, jurisdiction, administration and even time (e.g., slow to fast). While the global level of most scales is trivial to define, the local level of a scale is less clear. What becomes clear in the definition process between global and local, is the ambiguity and often arbitrary nature of defining what the reality of the system being examined is. Normative choices have to be made, especially for defining research questions and hypotheses, i.e., what is a part of the specific system, what is not considered to be a part of it, and what is an important element that you want to know about? This has implications for the relationships and outcomes you want to investigate. Standardization of system definitions, or system defining processes, would also be a useful consensus activity to increase comparability and exchange within system science. Entire fields of research have thus emerged around normative understandings of what the optimal system level unit to examine might be, such as rural sociology, landscape ecology, coastal governance or micro-economics. What is evident - and becomes unclear - is the spectrum of definitions and choices between the two ends of a scale, and the degree to which the categorical differences in system boundary definitions are meaningful for how we analyse and interpret their functionality.
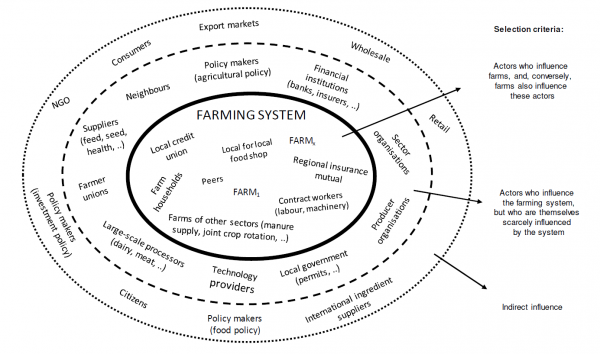
Defining System Boundaries
Out of these challenges to define system boundaries we recognize a clear gap on how to approach a globally coherent definition, which can recognize the wide array of contextual differences in how systems are defined and measured. We need to consider that some systems are either divided from larger or parallel systems, or that smaller systems are nested into other systems.
System boundaries can be defined based on a variety of parameters. For the simplicity of the argument, we focus on one parameter out of several, yet prefer not to discuss in depth the interaction of different parameters. Many people would define such interactions as complexity within systems thinking, but the examination of system complexity is premised on a coherent understanding of what is in and out of the system being examined. This is an inherent precondition for analysis, which is often discussed or taken as an assumption that is often not clearly defined. For example, spatially explicit parameters are an intuitive aspect of many defining processes, and therefore shape our perceptions of what an appropriate system boundary might be. To use a spatial example: a larger city can be divided into smaller neighbourhoods, while neighbourhoods can be defined based on different parameters, such as function, ethnic groups or cultural settings. While the definition of 'neighbourhoods' can be also informal, it is also well established within urban planning. There is an evident awareness that system boundaries exist, yet many would not be able to define why two systems differ. It is overall easier to define system boundaries based on spatial parameters, such as structural elements, ecosystems, or the design of a city, while informal parameters such as those based on normative dimensions are less clearly definable. Being initially clear within a research project about the boundaries of a system may help to clarify a ranking of what relevant parameters of the system are in terms of its boundaries, but also considering the variance of these parameters within a system. Basically, some parameters can be dramatically different within a system than outside, have no relevance within a system, or can have only relevance within the system. All assumptions are plausible parameters to serve as system boundaries.
Independent of the given parameter, we propose that both the state and the variance can be clear delimiters in defining boundaries between systems. When looking at a larger system, it can have an overall average value as well as an overall variance regarding a system parameter. If we would now divide the larger system into two smaller parts, it might be that the two systems have a different average value regarding this parameter. However, two smaller systems could also be different regarding their variance, where one smaller system has an overall large heterogeneity, and the other one a small heterogeneity.
A good example of this could be two neighbourhoods within one city. One could be very heterogeneous in terms of green infrastructure, having many smaller parks, trees, and green rooftops. The other one could be highly homogenous, being widely dominated by houses without any green infrastructure. Clearly, both systems vary both in terms of the average value, as well as the variance. Thus, where boundaries are drawn to define those neighbourhoods in that city will dictate the analysis and conclusions about its variance and values.
Another example would be two smaller systems, one again being highly heterogeneous, and the other consisting of one half that is a park, and another half that are large apartment houses. Many would opt to divide the second smaller system into two sub-systems. This example illustrates how different parameters allow for a different division of systems according to the habitat recognition. However, the park could be functionally related directly to the apartments, constituting its own unit in an urban planning context. This illustrates how system boundaries depend on the parameters we observe, but also the reasons why we want to measure them and what we want to know and value about the system.
More importantly, system boundaries can also differ regarding the average value of a parameter but can show different patterns when looking at the variance. This difference is often associated with the grain size of parameters. Grain size can be defined as the resolution at which a parameter is observed. A parameter can be for instance very homogenous at a large scale, yet very heterogenous at a small scale. The question of grain size is often associated with nestedness, yet grain size and nestedness are only indirectly connected, but not always necessarily linked.
For example, the functions of neighbourhood can be very homogenous at a coarse grain, yet heterogeneity is revealed at a more localized scale. This can be used to define the smallest local scale in a system, that is the scale at which the average as well as the variance of a parameter do not change any more. The smallest definable unit could hence be defined as the smallest local unit of a system. This is especially relevant since different disciplinary perspectives and approaches are often determined by method, and therefore often also by grain. While for instance a method might not find any relevant patterns based on one differentiation of sub-systems, another division might lead to meaningful patterns. This highlights why a clear determination of systems and their boundaries is so relevant.
System Boundaries and Normativity
Much of the currently published analysis in scientific publications is determined by system descriptions yet enabling transformation of systems demands a clear definition of the unit of analysis, i.e. a system, where common boundaries of those systems are not collectively agreed upon by all who are measuring and drawing conclusions about them. If a system being investigated is too large, relevant patterns of a parameter might be lost against its constituent internally contained parts. If a system is bound to small, again relevant patterns might be undetectable due to over-magnification.
This opens the door to criticism that could be raised regarding this line of thinking: Should the system boundaries be determined by the overall results that can be gained? From an inductive perspective, the answer could be different compared to a deductive perspective. Hence this question might be unsolvable for now due to the different epistemic preferences for trying to understand what is real through the scientific process. However, what can be said here is that Occam’s razor could apply. Every system should be as simple as possible, and as complex as necessary. This can be directly applied to the question of system boundaries, since these would clearly enable an overly simplistic or an overly complex analysis. While it is clear that system analysis cannot opt for trivial outcomes that are hard to understand, this highlights that there might be an optimal level and scale of analysis, which is probably closely tied to the relevance or ranking of the different parameters.
This is an important basis in order to enable the transformation of systems. The normative dimensions or parameters in systems are challenging to analyse and even more difficult to transform, yet building on two examples we show how the average as well as the variance of systems can allow for a clear analysis of how cultural difference can define system boundaries over time. For example, inheritance laws during the middle ages in Germany differed by religion. In Catholic regions, the eldest son inherited farms in rural areas; while in protestant areas, farms were divided into subsequent smaller units among the family. This had a dramatic impact on land use patterns as well as social dimensions within the given systems over time. Reflected in architecture and the landscape, these differences are often visible today, and can testify to how cultural variation can define systems functionality and boundaries.
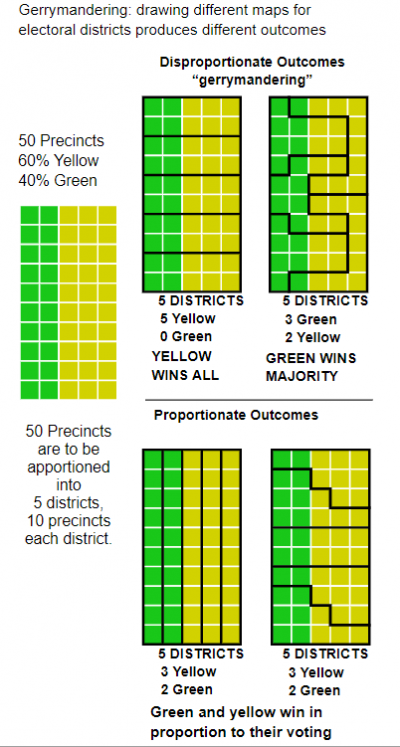
Another example is the process of political gerrymandering. This process depicts the manipulation of administrative boundaries for voting in the United States, with the goal to favour one political party over the other by drawing the lines around those neighbourhoods who will vote for your political party to be in the district, and those who won’t as out of it. This is a prominent example of how through the altering of system boundaries the normative characteristics of a system are altered. This is done with the rationale to diminish the variance in terms of some factors, for instance with the goal to represent a minority. De facto, is this rational often misused to influence the average, or in other words to construct majorities. This highlights how system boundaries are already actively recognized, even on such a deep normative dimension such as the political value system.
Hence system borders can extend beyond biophysical spatial system entities and can also include the difference in mindsets and cultural settings. The most important question when defining system boundaries then becomes, which variables determine or influence the system dynamics the most. When trying to define system boundaries, we are hence looking at a multiverse of multidimensional parameters. We of course acknowledge the interactions between these parameters - which is often coined as complexity - but let us not discuss this further at this point.
Instead we conclude by shortly discussing the importance of a ranking or the development of hierarchies of different system parameters to guide systems analysis. Some parameters might be more important than others regarding the dynamics within one system. On the other hand, some parameters may be more important in one system than in others. Based on these two assumptions, any definition of system boundaries, which can be defined as an early step in systems analysis and transformation, can be approaches as an iterative approach. Ideally, when defining system boundaries, we would propose a parsimonious approach, which we define as being as simple as possible and as complex as necessary.
In an example where three systems neighbour each other, two systems (S1 and S2) could share a larger number of parameters that are important for the dynamics of these two systems. If then one of these two (S1) shared a strong overlap with a third system (S3), and these were strongly explained by only one parameter, we should prioritize the declaration of S1 and S3 as more similar. Consequently, S1 and S3 would be declared as sharing a less strong boundary than systems S1 and S2. However, if system S2 and S3 share no parameters that explain their system dynamics, then the boundary between S2 and S3 is the strongest. These types of boundaries, can be spatially, but quite often they can also manifest in forms of social values, economic barriers and in other phenomena.
This example underlines the importance of defining system boundaries by similarities and dissimilarities, which can be defined both by the average value of parameters, as well as by the variance of parameters. The additional layer of the social value we assign to those parameters then provides a means by which we can make analytical choices based on how we view order such parameters into value hierarchies. We propose that instead of relying on informal or non-reproducible definitions of system boundaries, it is vital to pre-analyse system dynamics to define system boundaries, which are a pre-condition for a proper analysis of system dynamics. Otherwise a system analysis will lack the preconditions necessary that enable its meaning and implications to be understood ad compared with other systems.
The author of this entry is Henrik von Wehrden.