Difference between revisions of "Agency, Complexity and Emergence"
(38 intermediate revisions by 6 users not shown) | |||
Line 1: | Line 1: | ||
− | + | '''Note:''' The German version of this entry can be found here: [[Agency, Complexity and Emergence (German)]] | |
− | + | ||
+ | '''In short:''' This entry discusses three central elements of systems, and how we can investigate them methodologically. | ||
+ | |||
+ | Agency, Complexity and Emergence are three concepts that can be understood as lenses. Through them, we can see results that originate in theoretical considerations, as well as information we access through empirical research. Since such [[Glossary|concepts]] are '''binding the two realms of scientific inquiry - theory and practice - I call the three concepts of agency, complexity and emergence „boundary objects“.''' They are good examples of ideas that are most relevant within the philosophy of science. In addition, they strongly determine our fundamental and normative view of the world as well as the consequences of the knowledge we gain from the world. If I have for instance the world view that no free will exists, then this will also be a fundamental influence on how I interpret results from empirical inquiry of the actions of people. Many would agree that people have the potential to have free will, just as all milk can be turned into butter. There are other concepts besides agency, complexity and emergence that are worth pursuing form the perspective of philosophy of science, but for the sake of simplicity and priority, [[Big problems for later|we will only deal]] with these three boundary object here. Let us start with an attempt to show the '''diversity of definitions of these concepts'''. | ||
+ | |||
== Agency == | == Agency == | ||
− | The most simple definition of agency that is widely considered to be most relevant from a methodological standpoint is that agency is defined as the capacity of an individual to act intentionally with the assumption of a causal outcome based on this action. However, within different discourses and disciplines there is a wide variety of approaches that consider broader or more narrow definitions of agency. There is a first disagreement whether an agent should have a mental state that is able to anticipate the outcome of the action, and such diverse realms as neuroscience and philosophy disagree on that point already. For instance, reflexes are instincts, and it is very difficult to track down whether such actions are intentional and contain assumptions about the outcome. Therefore, broader definitions of agency include the action-based adaptation of an agent to | + | The most simple definition of agency that is widely considered to be most relevant from a methodological standpoint is that agency is defined as the following: ''the capacity of an individual to act intentionally with the assumption of a causal outcome based on this action''. However, within different discourses and disciplines there is a wide variety of approaches that consider broader or more narrow definitions of agency. There is a first disagreement whether an agent should have a mental state that is able to anticipate the outcome of the action, and such diverse realms as neuroscience and philosophy disagree on that point already. For instance, reflexes are instincts, and it is very difficult to track down whether such actions are intentional and contain assumptions about the outcome. Therefore, broader definitions of agency include the action-based adaptation of an agent to their or its environment, which includes also much of the [https://plato.stanford.edu/entries/moral-animal/ non-human animal kingdom]. This broad definition of agency will be ignored here, although it should be stated that the actions of some higher non-human animals indicate intention and mental states that may be able to anticipate. These are however difficult to investigate from a methodological standpoint, although much can be expected from this direction in the future. |
+ | [[File:Tiger.jpg.jpg|350px|thumb|right|'''Non-human animals react to their environment, but do they also act intentionally?''' Source: pixabay]] | ||
+ | '''What is relevant to consider is that actions of agents need to be wilful, i.e. a mere act that can be seen as serendipity is not part of agency.''' Equally, non-anticipated consequences of actions based on causal chains are a problem in agency. Agency is troubled when it comes to either acknowledging serendipity, or Murphy's law. Such lucky or unlucky actions were not anticipated by the agents, and are therefore not really included in the definition of agency. There is thus a metaphysical problem when we try to differentiate the agent, their actions, and the consequences of their actions. One could claim that this can be solved by focussing on the consequences of the actions of agents alone. However, this consequentialist view is partly a theoretical consideration, as this view can create many interesting experiments, but does not really help us to solve the problem of unintentional acts per se. Still, consequentialism and a focus on mere actions is also relevant because it allows to step away from a single agent and focus instead on the interactions within the agency of several actors, which is a good start for the next concept. | ||
− | |||
== Complexity == | == Complexity == | ||
− | Many phenomena in nature are simple, and follow simple rules. Many other phenomena are not simple, but instead can be defined as "complex". In order to negotiate between the two there is the fundamental law of Occam's razor, which defines that all things | + | Many phenomena in nature are simple, and follow simple rules. Many other phenomena are not simple, but instead can be defined as "complex". In order to negotiate between the two there is the fundamental law of Occam's razor, which defines that all things should be as simple as possible, and as complex as necessary. Complex system theory strongly developed over the last decades, and created ripples into all empirical science and beyond. '''What is however problematic is that no unified and universally accepted definition of complexity exists, which is quite ironic.''' It seems that complexity in itself is complex. Here, I will therefore focus on some of the main arguments and characteristics that are relevant from a methodological standpoint. First of all, I will restrict all considerations to 'complex systems'. To this end, I simply define [[System Thinking & Causal Loop Diagrams|„systems“]] as any number of individuals or elements that interact. Complex Systems, then, are systems that are composed of many components which may interact with each other in various ways and which are therefore difficult to model. Specific properties include [[Glossary|non-linearity]], emergence, adaptation and [[Glossary|feedback loops]]. From a methodological standpoint we should be able to observe these interactions, while from a philosophical standpoint we should be able to reflect upon them. For more background on the definition of System Boundaries, please refer to [[System Boundaries|this entry.]] |
+ | |||
+ | [[File:System.jpg.jpg|350px|thumb|left|'''Systems can become quite large, and their interaction may lead to complex dynamics.''' Source: pixabay]] | ||
+ | Such interactions can be simply adaptive, which is a key characteristic of many organisms and systems, but we may not be able to anticipate such adaptations. Through interactions, networks may form that can be observed, yet not be anticipated. Interactions can create feedback mechanisms or actions that reinforce [[Glossary|patterns]], creating what many call 'complex interactions'. I would argue that these interactions become often unpredictable because they exceed our original assumptions about the dynamics that are possible in the given system. The complexity of such dynamics is then rooted in our own imperfect assumptions about such system dynamics. | ||
+ | |||
+ | Another phenomenon in complex systems is the question of non-linearity. Non-linear behavior is - mechanically speaking - changes that occur strongly and suddenly at the same time, and not gradually and continuously. Non-linear dynamics in systems are often occurring because the dynamics we observe are mediated by another factor. This additional and unknown factor or variable is the original trigger that in interaction with other parts of the system leads to non-linear system dynamics. The third characteristic in complex systems is related to spontaneous order. Spontaneous order relates to "the spontaneous emergence of order out of seeming chaos" (wikipedia), i.e. the creation of structures and order where it could not be anticipated. An example is the synchronisation of clapping noises in a crowd. Such spontaneous orders can often not be anticipated, and provide thus a problem when considering agency of systems. A lack of possible anticipation of feedback loops and non-linear behavior can thus lead to a spontaneous order that highlights our own imperfections when understanding systems. Such system states can be called emergent. | ||
+ | |||
== Emergence == | == Emergence == | ||
− | If two or more entities together have a characteristic or behaviour that could not be anticipated only based on their individual parts, then this non-anticipated property is called "emergent". '''To me, a good example of emergence are the Beatles.''' The Beatles were much more than just John, Paul, George and Ringo. Another prominent examples is water, which can extinguish fire. Oxygen and hydrogen do quite the opposite with fire, they propel it. These two examples show one of the key problems of emergence: it is quite simple to find examples for it, yet it is very hard to find underlying laws | + | [[File:The Beatles.jpg.jpg|350px|thumb|right|'''What defined the Beatles was certainly more than the sum of their band members.''' Source: pixabay]] |
+ | If two or more entities together have a characteristic or behaviour that could not be anticipated only based on their individual parts, then this non-anticipated property is called "emergent". '''To me, a good example of emergence are the Beatles.''' The Beatles were much more than just John, Paul, George and Ringo. Another prominent examples is water, which can extinguish fire. Oxygen and hydrogen do quite the opposite with fire, they propel it. These two examples show one of the key problems of emergence: it is quite simple to find examples for it, yet it is very hard to find underlying laws or even lines of thinking that can be used to approximate principles of emergence. Again, emergence is - just as complexity - a fluid if not untamable concept in itself. Empirical researchers are fascinated when they encounter it, yet it cannot be solved in itself, because that is what emergence is all about. While I will [[Big problems for later|leave this to philosophy]], one might argue that some things are not meant to be anticipated. Humans today are unlocking ever more strata of reality which has allowed us to capture many emergent phenomena, such as quantum physics, organic chemistry, behaviour biology and many higher system dynamics. All emergent phenomena build on interconnectedness of the parts that interact and thus jointly emerge. Another relevant criterion is defined as fallacy of division - parts of a whole may show differing behaviour than the whole. This is again related to interconnectedness, but also to the variance that might exist within a population. Many phenomena of the natural world show patterns of emergence, such as the physics of sand. Chemical reactions, biological interactions and our economical system in itself are all emergent. Yet, if we cannot predict them, how can we empirically approach them? | ||
== Methodological implications == | == Methodological implications == | ||
− | Considering these three | + | Considering these three concepts, from a methodological standpoint we should consequently ask which entities lack agency, which systems are simple, and which interconnected phenomena defy any emergence. Falsification is one of the most important principles of science, and applying this approach to these three concepts can help us approximate the current methodological frontier in science. The word "current" is of central importance here, because we have to understand that all three concepts are at least partly normative, because they revolve around our current state of knowledge. Individuals that we deny agency now may be attributed agency by our grid of theory and methods at some point. Systems that seem complex today may be simple in the future. Emergent phenomena today may enable predictability of future interconnections. '''To this end, these three concepts are merely snapshots of our own ignorance'''. Right now we cannot know whether in the very long run all agency will be tracked, all systems will be simple, and all interconnections predictable, which right now only philosophy can examine. I think empirical research right now is incapable to answer how much people in the future may be able to understand, but it is not for us to decide how much will be explained in the future. |
What is clearer is that ''agency'' widely revolves around complex experimental designs that bridge different realms of science, such as neuroscience and psychology, psychology and microeconomics or behavioural science and zoology. The concept of agency links different fields of inquiry. Besides our diverse theories when it comes to agency, we only start to fully acknowledge the challenges related to its measurement as well as the opportunities to bridge different domains of sciences. From an empirical standpoint, agency has not been systematically accessed, which is why much emphasis is given to some few studies, and existing schools of thought often build on diverse premises. A good example to access agency is for instance in the combination of psychological or behaviour focused experiments with neurological examinations, although even such complicated experimental settings are not free of ambiguous results. Another methodological approach to agency could be in gamification, since such approaches would be able to test behavior of players within a complex environment, including reactions and motivations. The concept of altruism does however illustrate that, for the time being, the explanation of such behaviors may be unattainable. Just like agency, altruism can be divided into evolutionary or metaphysical explanations, roughly speaking. Time will tell if these schools of thought can be bridged. | What is clearer is that ''agency'' widely revolves around complex experimental designs that bridge different realms of science, such as neuroscience and psychology, psychology and microeconomics or behavioural science and zoology. The concept of agency links different fields of inquiry. Besides our diverse theories when it comes to agency, we only start to fully acknowledge the challenges related to its measurement as well as the opportunities to bridge different domains of sciences. From an empirical standpoint, agency has not been systematically accessed, which is why much emphasis is given to some few studies, and existing schools of thought often build on diverse premises. A good example to access agency is for instance in the combination of psychological or behaviour focused experiments with neurological examinations, although even such complicated experimental settings are not free of ambiguous results. Another methodological approach to agency could be in gamification, since such approaches would be able to test behavior of players within a complex environment, including reactions and motivations. The concept of altruism does however illustrate that, for the time being, the explanation of such behaviors may be unattainable. Just like agency, altruism can be divided into evolutionary or metaphysical explanations, roughly speaking. Time will tell if these schools of thought can be bridged. | ||
− | ''Complex system theory'' is increasingly in the focus of many fields of research, which is no surprise. The dynamics of complex systems are an Eldorado for many empirical researchers, and many disciplines are engaged in this arena. From a methodological standpoint, methods such as network analysis or structural equation models and also theoretical work such as the [https://en.wikipedia.org/wiki/Elinor_Ostrom Ostrom framework] are examples of the increasing recognition of complex systems. The Ostrom framework builds a bridge to this end, as it links a conceptual focus on resources with a methodological line of thinking to allow for a structured recognition of system dynamics. Several approaches try to implement the dynamics of complex systems within an analytical framework, yet these approaches often suffer from a gap between empirical data and sufficiently complex theory-driven models, since we only start to approach the gap between individual case studies and a broader view of empirical results. Here, the Ostrom framework is recognized as a landmark approach, although we must understand that even this widely popular framework was implemented in surprisingly few empirical studies (Partelow 2019). | + | ''Complex system theory'' is increasingly in the focus of many fields of research, which is no surprise. The dynamics of [[Glossary|complex systems]] are an Eldorado for many empirical researchers, and many disciplines are engaged in this arena. From a methodological standpoint, methods such as network analysis or structural equation models and also theoretical work such as the [https://en.wikipedia.org/wiki/Elinor_Ostrom Ostrom framework] are examples of the increasing recognition of complex systems. The Ostrom framework builds a bridge to this end, as it links a conceptual focus on resources with a methodological line of thinking to allow for a structured recognition of system dynamics. Several approaches try to implement the dynamics of complex systems within an analytical [[Glossary|framework]], yet these approaches often suffer from a gap between empirical data and sufficiently complex theory-driven models, since we only start to approach the gap between individual case studies and a broader view of empirical results. Here, the Ostrom framework is recognized as a landmark approach, although we must understand that even this widely popular framework was implemented in surprisingly few empirical studies (Partelow 2019). |
+ | [[File:Ostrom framework.jpg|400px|thumb|left|'''The Ostrom framework allows us to reflect on socio-ecological systems.''' Source: [https://science.sciencemag.org/content/325/5939/419.abstract Ostrom 2009.]]] | ||
To this end, it is important to realise that the problems that complex systems may encompass can be often diagnosed - which is called system knowledge - however the transformative knowledge necessary to solve these problems is ultimately what is complex. For instance do many people know that we already understood that climate change is real, and what we could do against it. What we could really do to solve the deeper problems - i.e. changing people's behaviour and consumption patterns - is however much more difficult to achieve, and this is what complex system thinking often about. '''Problem diagnosis is often simpler than creating solutions.''' Another challenge in complex systems are temporal dynamics. For instance, meteorological models are built on complex theories yet are also constantly refined based on a diversity of data. These models showcase that we are able to predict the weather for some days, and coarse patterns even for weeks, but there is still a clear limitation of our understanding of the weather when we go further into the future. A recent example of complex system dynamics is the Corona pandemic. While in the early stage of the pandemic our knowledge about the spread of the virus and the associated dynamics grew quickly, effective solutions are a long-term goal. There are clear relations how certain actions lead to certain developments in the pandemic, but the local and regional context can cascade into severe dynamics and differences. In addition, the development of potential solutions - such as a vaccine - is very complex and difficult to achieve. While thus many developments during this pandemic can be understood, it is certainly more complex to create a solution. | To this end, it is important to realise that the problems that complex systems may encompass can be often diagnosed - which is called system knowledge - however the transformative knowledge necessary to solve these problems is ultimately what is complex. For instance do many people know that we already understood that climate change is real, and what we could do against it. What we could really do to solve the deeper problems - i.e. changing people's behaviour and consumption patterns - is however much more difficult to achieve, and this is what complex system thinking often about. '''Problem diagnosis is often simpler than creating solutions.''' Another challenge in complex systems are temporal dynamics. For instance, meteorological models are built on complex theories yet are also constantly refined based on a diversity of data. These models showcase that we are able to predict the weather for some days, and coarse patterns even for weeks, but there is still a clear limitation of our understanding of the weather when we go further into the future. A recent example of complex system dynamics is the Corona pandemic. While in the early stage of the pandemic our knowledge about the spread of the virus and the associated dynamics grew quickly, effective solutions are a long-term goal. There are clear relations how certain actions lead to certain developments in the pandemic, but the local and regional context can cascade into severe dynamics and differences. In addition, the development of potential solutions - such as a vaccine - is very complex and difficult to achieve. While thus many developments during this pandemic can be understood, it is certainly more complex to create a solution. | ||
− | Tracking down ''emergence'' has become a key focus in many areas of science, but organic chemistry can serve as an example of how much has been done, yet how much is still on the horizon. Many negative effects of chemicals were not anticipated, with prominent examples being FCKW, pesticides and antibiotics. The effect of different medical drugs on people is yet another example, since interactions between different medications are hardly understood at all, as the field only slowly unravels the negative side-effects of interacting medications or treatments. We are far away from understanding the impact that the concepts ''agency, complex systems'' and ''emergence'' have on our knowledge. Yet, we need to diversify our canon of methods in order to approach these concepts from an empirical standpoint. Otherwise we will not be able to unlock new strata of knowledge. This includes the combination of different methods, the utilization of specific methods in a different context, as well as the development of novel methods. | + | Tracking down ''emergence'' has become a key focus in many areas of science, but organic chemistry can serve as an example of how much has been done, yet how much is still on the horizon. Many negative effects of chemicals were not anticipated, with prominent examples being FCKW, pesticides and antibiotics. The effect of different medical drugs on people is yet another example, since interactions between different medications are hardly understood at all, as the field only slowly unravels the negative side-effects of interacting medications or treatments. We are far away from understanding the impact that the concepts ''agency'', ''complex systems'' and ''emergence'' have on our knowledge. Yet, we need to diversify our canon of methods in order to approach these concepts from an empirical standpoint. Otherwise we will not be able to unlock new strata of knowledge. This includes the combination of different methods, the utilization of specific methods in a different context, as well as the development of novel methods. |
− | What is however clear is that the three concepts - agency, complexity and emergence - have consequences about our premises of empirical knowledge. What if ultimately nothing is generalisable? What if all valid arguments are only valid for a certain time? And what if some strata will forever escape a truly reliable measurement? We [[Big problems for later|cannot answer]] these problems here, yet it is important to differentiate what we know, what we may be able to know, and what we will probably never know. The [https://www.britannica.com/science/uncertainty-principle uncertainty principle of Heisenberg] in Quantum mechanics which refers to the the position and momentum of particles illustrates that some things cannot be approximated, observed or known. Equal claims can be made about larger phenomena, such as personal identity. Hence, as much as agency, complex systems and emergence can be boundary objects for methods, they equally highlight our (current) limitations. | + | What is however clear is that the three concepts - agency, complexity and emergence - have consequences about our premises of empirical knowledge. What if ultimately nothing is generalisable? What if all valid arguments are only valid for a certain time? And what if some strata will forever escape a truly reliable measurement? We [[Big problems for later|cannot answer]] these problems here, yet it is important to differentiate what we know, what we may be able to know, and what we will probably never know. The [https://www.britannica.com/science/uncertainty-principle uncertainty principle of Heisenberg] in Quantum mechanics which refers to the the position and momentum of particles illustrates that some things cannot be approximated, observed or known. Equal claims can be made about larger phenomena, such as [https://www.abc.net.au/listen/programs/philosopherszone/personal-identity:-a-primer/8996320 personal identity]. Hence, as much as agency, complex systems and emergence can be boundary objects for methods, they equally highlight our (current) limitations. |
== The way forward == | == The way forward == | ||
− | If we want to empirically investigate agency, we first and foremost investigate individuals, or actions of entities | + | If we want to empirically investigate agency, we first and foremost investigate individuals, or actions of homogeneous entities, or consequences of actions of individuals. All this has consequences for the methods we apply, and the questions whether we observe or test premises has in addition further methodologial ramifications. '''I can interview individuals, yet this will hardly allow me to prove agency.''' Because of this, much of our current knowledge of agency is either rooted in widely deductive testing of very clear hypotheses, or questions of either logic or metaphysics, which are mostly associated with philosophy. |
+ | |||
+ | The investigation of complex systems has thrived in the last decades, both from an empirical as well as from a conceptual perspective. Many methods emerged or were subsequently adapted to answer questions as well as explore relations, and this thrive towards a deeper understanding of systems is at least one important difference to agency from a methodological standpoint. Much novel data is available, and often inductively explored. The scale of complex systems makes an intervention with a focus on [[Causality and correlation|causality]] a challenge, hence many investigated relations are purely correlative. Take for instance social media, or economic flows, which can be correlatively investigated, yet causality is an altogether different matter. This creates a methodological challenge concerning the fundamental assumptions that govern the interpretations of scientists. Many of our scientific questions are normative and many researchers assume causality in their investigations. This is at least partly because they are part of a discipline or tradition that assumes causality, and this makes them part of a normative group of scientists following this assumption. While this is trivial in itself, it illustrates that simply being part of a group of scientists that assumes causality cannot be a valid argument to actually confirm a causal relation. These two notions differ. | ||
+ | |||
+ | Another methodological problem related to causality are non-linear relations, since much of the statistical canon is based on probability and linear relations. While linear relations allow for a better inference of causal explanations, the long existing yet until recently hardly explored [[Bayesian inference|Bayesian]] statistics are an example that we can inductively learn about systems at a growing pace without being dependent on linearity or normal-distributions. This Bayesian revolution is currently under way, but much of the disciplines relying on statistics did not catch up on this yet. Other methodological approaches will certainly be explored to gain insight into the nuts and bolts of complex systems, yet this is only slowly emerging. | ||
+ | |||
+ | The whole globe - although not a closed system – can be seen as a global system, and this is certainly worthwhile pursuing from a methodological standpoint. Still, global dynamics consist of such diverse data, that simply the translational act to bring different data together seems almost impossible right now. While emergence can lead to novel solutions, globalisation and technology have triggered uncountable events of emergence, such as global conflicts, climate change, increase in cancer rates and biodiversity loss. Humankind did certainly not plan these potential endpoints of ourselves, instead they emerged out of unpredictable combinations of our actions. From a methodological standpoint, these events are just as unpredictable as is the effect which two molecules have onto each other and the environment. '''Emergence is a truly cross-scalar phenomenon.''' Consequently, many methodological accounts to counteract threats to human societies are correlative if they are empirical. We are far away from any deep understanding of emergence, and what makes phenomena emergent. | ||
+ | [[File:Climate model.png|400px|thumb|right|'''Climate models are increasingly getting more accurate, but the complexity and emergence of the global climate system may never be fully understood.''' Source: [https://blogs.egu.eu/geolog/2018/09/19/how-to-forecast-the-future-with-climate-models/ European Geosciences Union]]] | ||
− | + | Based on the current methodological canon we can say: '''Much is not (yet?) observed, much is not known, and it will be crucial to understand what we will not be able to know, at least for the time being.''' These three different qualities of knowledge clearly highlight that research will have to collaborate - agency, many complex systems and several phenomena that are emergent cannot be sufficiently investigated by one discipline alone. While chemistry may find emergence in chemical reactions, and medicine may find interactions between different drugs, these results become normative as soon as they are applicable in the real world. To this claim, one could make the exception of ethics that are inherently normative. More broadly speaking, only philosophy can meaningfully engage with all three domains of knowledge - unobserved, not known and never known - yet may not be able to contribute to all empirical problems. This means, everything except philosophy may be able to go just into two of the three realms, the un-observed and unknown. We need to combine our methodological approaches to create the knowledge that is needed now. The need for collaboration is a question of responsibility, and only if all disciplines dissolve (or at least redefine their main goal to unite and not differentiate), agency may be fully explored and complex problems may be solved. Many of the solutions we have in our hands right now already would seem like wizardry to our ancestors. It is our responsibility to continue on this path, not as technocrats or positivists that have arrogant pride of their achievements, but as mere contributors to a wider debate that should ultimately encompass all society. | |
− | + | To say it with Derek Parfit: | |
− | + | "Some things (...) matter, and there are better and worse way to live. After many thousands of years of responding to reasons in ways that helped them to survive and reproduce, human beings can now respond to other reasons. We are a part of a Universe that is starting to understand itself. And we can partly understand, not only what is in fact true, but also what ought to be true, and what we might be able to make true. What now matters the most is that we avoid ending human history. If there are no rational beings elsewhere, it may depend on us and our successors whether it will all be worth it, because the existence of the Universe will have been on the whole good." | |
− | + | == Further Information == | |
+ | * A [https://www.youtube.com/watch?v=16W7c0mb-rE video by Kurzgesagt] that illustrates the concept of Emergence | ||
+ | * Calarco, Matthew. 2015. "Thinking through Animals - Identity, Difference, Indistinction" (An introduction to Critical Animal Studies to counter human-centered viewpoints) | ||
+ | * Parfit, Derek. 1984. "Reasons and Persons" | ||
+ | ---- | ||
+ | [[Category: Normativity of Methods]] | ||
− | + | The [[Table_of_Contributors|author]] of this entry is Henrik von Wehrden. |
Latest revision as of 14:28, 15 January 2024
Note: The German version of this entry can be found here: Agency, Complexity and Emergence (German)
In short: This entry discusses three central elements of systems, and how we can investigate them methodologically.
Agency, Complexity and Emergence are three concepts that can be understood as lenses. Through them, we can see results that originate in theoretical considerations, as well as information we access through empirical research. Since such concepts are binding the two realms of scientific inquiry - theory and practice - I call the three concepts of agency, complexity and emergence „boundary objects“. They are good examples of ideas that are most relevant within the philosophy of science. In addition, they strongly determine our fundamental and normative view of the world as well as the consequences of the knowledge we gain from the world. If I have for instance the world view that no free will exists, then this will also be a fundamental influence on how I interpret results from empirical inquiry of the actions of people. Many would agree that people have the potential to have free will, just as all milk can be turned into butter. There are other concepts besides agency, complexity and emergence that are worth pursuing form the perspective of philosophy of science, but for the sake of simplicity and priority, we will only deal with these three boundary object here. Let us start with an attempt to show the diversity of definitions of these concepts.
Contents
Agency
The most simple definition of agency that is widely considered to be most relevant from a methodological standpoint is that agency is defined as the following: the capacity of an individual to act intentionally with the assumption of a causal outcome based on this action. However, within different discourses and disciplines there is a wide variety of approaches that consider broader or more narrow definitions of agency. There is a first disagreement whether an agent should have a mental state that is able to anticipate the outcome of the action, and such diverse realms as neuroscience and philosophy disagree on that point already. For instance, reflexes are instincts, and it is very difficult to track down whether such actions are intentional and contain assumptions about the outcome. Therefore, broader definitions of agency include the action-based adaptation of an agent to their or its environment, which includes also much of the non-human animal kingdom. This broad definition of agency will be ignored here, although it should be stated that the actions of some higher non-human animals indicate intention and mental states that may be able to anticipate. These are however difficult to investigate from a methodological standpoint, although much can be expected from this direction in the future.
What is relevant to consider is that actions of agents need to be wilful, i.e. a mere act that can be seen as serendipity is not part of agency. Equally, non-anticipated consequences of actions based on causal chains are a problem in agency. Agency is troubled when it comes to either acknowledging serendipity, or Murphy's law. Such lucky or unlucky actions were not anticipated by the agents, and are therefore not really included in the definition of agency. There is thus a metaphysical problem when we try to differentiate the agent, their actions, and the consequences of their actions. One could claim that this can be solved by focussing on the consequences of the actions of agents alone. However, this consequentialist view is partly a theoretical consideration, as this view can create many interesting experiments, but does not really help us to solve the problem of unintentional acts per se. Still, consequentialism and a focus on mere actions is also relevant because it allows to step away from a single agent and focus instead on the interactions within the agency of several actors, which is a good start for the next concept.
Complexity
Many phenomena in nature are simple, and follow simple rules. Many other phenomena are not simple, but instead can be defined as "complex". In order to negotiate between the two there is the fundamental law of Occam's razor, which defines that all things should be as simple as possible, and as complex as necessary. Complex system theory strongly developed over the last decades, and created ripples into all empirical science and beyond. What is however problematic is that no unified and universally accepted definition of complexity exists, which is quite ironic. It seems that complexity in itself is complex. Here, I will therefore focus on some of the main arguments and characteristics that are relevant from a methodological standpoint. First of all, I will restrict all considerations to 'complex systems'. To this end, I simply define „systems“ as any number of individuals or elements that interact. Complex Systems, then, are systems that are composed of many components which may interact with each other in various ways and which are therefore difficult to model. Specific properties include non-linearity, emergence, adaptation and feedback loops. From a methodological standpoint we should be able to observe these interactions, while from a philosophical standpoint we should be able to reflect upon them. For more background on the definition of System Boundaries, please refer to this entry.
Such interactions can be simply adaptive, which is a key characteristic of many organisms and systems, but we may not be able to anticipate such adaptations. Through interactions, networks may form that can be observed, yet not be anticipated. Interactions can create feedback mechanisms or actions that reinforce patterns, creating what many call 'complex interactions'. I would argue that these interactions become often unpredictable because they exceed our original assumptions about the dynamics that are possible in the given system. The complexity of such dynamics is then rooted in our own imperfect assumptions about such system dynamics.
Another phenomenon in complex systems is the question of non-linearity. Non-linear behavior is - mechanically speaking - changes that occur strongly and suddenly at the same time, and not gradually and continuously. Non-linear dynamics in systems are often occurring because the dynamics we observe are mediated by another factor. This additional and unknown factor or variable is the original trigger that in interaction with other parts of the system leads to non-linear system dynamics. The third characteristic in complex systems is related to spontaneous order. Spontaneous order relates to "the spontaneous emergence of order out of seeming chaos" (wikipedia), i.e. the creation of structures and order where it could not be anticipated. An example is the synchronisation of clapping noises in a crowd. Such spontaneous orders can often not be anticipated, and provide thus a problem when considering agency of systems. A lack of possible anticipation of feedback loops and non-linear behavior can thus lead to a spontaneous order that highlights our own imperfections when understanding systems. Such system states can be called emergent.
Emergence
If two or more entities together have a characteristic or behaviour that could not be anticipated only based on their individual parts, then this non-anticipated property is called "emergent". To me, a good example of emergence are the Beatles. The Beatles were much more than just John, Paul, George and Ringo. Another prominent examples is water, which can extinguish fire. Oxygen and hydrogen do quite the opposite with fire, they propel it. These two examples show one of the key problems of emergence: it is quite simple to find examples for it, yet it is very hard to find underlying laws or even lines of thinking that can be used to approximate principles of emergence. Again, emergence is - just as complexity - a fluid if not untamable concept in itself. Empirical researchers are fascinated when they encounter it, yet it cannot be solved in itself, because that is what emergence is all about. While I will leave this to philosophy, one might argue that some things are not meant to be anticipated. Humans today are unlocking ever more strata of reality which has allowed us to capture many emergent phenomena, such as quantum physics, organic chemistry, behaviour biology and many higher system dynamics. All emergent phenomena build on interconnectedness of the parts that interact and thus jointly emerge. Another relevant criterion is defined as fallacy of division - parts of a whole may show differing behaviour than the whole. This is again related to interconnectedness, but also to the variance that might exist within a population. Many phenomena of the natural world show patterns of emergence, such as the physics of sand. Chemical reactions, biological interactions and our economical system in itself are all emergent. Yet, if we cannot predict them, how can we empirically approach them?
Methodological implications
Considering these three concepts, from a methodological standpoint we should consequently ask which entities lack agency, which systems are simple, and which interconnected phenomena defy any emergence. Falsification is one of the most important principles of science, and applying this approach to these three concepts can help us approximate the current methodological frontier in science. The word "current" is of central importance here, because we have to understand that all three concepts are at least partly normative, because they revolve around our current state of knowledge. Individuals that we deny agency now may be attributed agency by our grid of theory and methods at some point. Systems that seem complex today may be simple in the future. Emergent phenomena today may enable predictability of future interconnections. To this end, these three concepts are merely snapshots of our own ignorance. Right now we cannot know whether in the very long run all agency will be tracked, all systems will be simple, and all interconnections predictable, which right now only philosophy can examine. I think empirical research right now is incapable to answer how much people in the future may be able to understand, but it is not for us to decide how much will be explained in the future.
What is clearer is that agency widely revolves around complex experimental designs that bridge different realms of science, such as neuroscience and psychology, psychology and microeconomics or behavioural science and zoology. The concept of agency links different fields of inquiry. Besides our diverse theories when it comes to agency, we only start to fully acknowledge the challenges related to its measurement as well as the opportunities to bridge different domains of sciences. From an empirical standpoint, agency has not been systematically accessed, which is why much emphasis is given to some few studies, and existing schools of thought often build on diverse premises. A good example to access agency is for instance in the combination of psychological or behaviour focused experiments with neurological examinations, although even such complicated experimental settings are not free of ambiguous results. Another methodological approach to agency could be in gamification, since such approaches would be able to test behavior of players within a complex environment, including reactions and motivations. The concept of altruism does however illustrate that, for the time being, the explanation of such behaviors may be unattainable. Just like agency, altruism can be divided into evolutionary or metaphysical explanations, roughly speaking. Time will tell if these schools of thought can be bridged.
Complex system theory is increasingly in the focus of many fields of research, which is no surprise. The dynamics of complex systems are an Eldorado for many empirical researchers, and many disciplines are engaged in this arena. From a methodological standpoint, methods such as network analysis or structural equation models and also theoretical work such as the Ostrom framework are examples of the increasing recognition of complex systems. The Ostrom framework builds a bridge to this end, as it links a conceptual focus on resources with a methodological line of thinking to allow for a structured recognition of system dynamics. Several approaches try to implement the dynamics of complex systems within an analytical framework, yet these approaches often suffer from a gap between empirical data and sufficiently complex theory-driven models, since we only start to approach the gap between individual case studies and a broader view of empirical results. Here, the Ostrom framework is recognized as a landmark approach, although we must understand that even this widely popular framework was implemented in surprisingly few empirical studies (Partelow 2019).
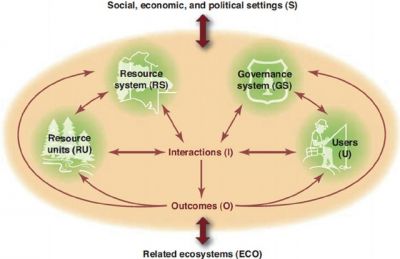
To this end, it is important to realise that the problems that complex systems may encompass can be often diagnosed - which is called system knowledge - however the transformative knowledge necessary to solve these problems is ultimately what is complex. For instance do many people know that we already understood that climate change is real, and what we could do against it. What we could really do to solve the deeper problems - i.e. changing people's behaviour and consumption patterns - is however much more difficult to achieve, and this is what complex system thinking often about. Problem diagnosis is often simpler than creating solutions. Another challenge in complex systems are temporal dynamics. For instance, meteorological models are built on complex theories yet are also constantly refined based on a diversity of data. These models showcase that we are able to predict the weather for some days, and coarse patterns even for weeks, but there is still a clear limitation of our understanding of the weather when we go further into the future. A recent example of complex system dynamics is the Corona pandemic. While in the early stage of the pandemic our knowledge about the spread of the virus and the associated dynamics grew quickly, effective solutions are a long-term goal. There are clear relations how certain actions lead to certain developments in the pandemic, but the local and regional context can cascade into severe dynamics and differences. In addition, the development of potential solutions - such as a vaccine - is very complex and difficult to achieve. While thus many developments during this pandemic can be understood, it is certainly more complex to create a solution.
Tracking down emergence has become a key focus in many areas of science, but organic chemistry can serve as an example of how much has been done, yet how much is still on the horizon. Many negative effects of chemicals were not anticipated, with prominent examples being FCKW, pesticides and antibiotics. The effect of different medical drugs on people is yet another example, since interactions between different medications are hardly understood at all, as the field only slowly unravels the negative side-effects of interacting medications or treatments. We are far away from understanding the impact that the concepts agency, complex systems and emergence have on our knowledge. Yet, we need to diversify our canon of methods in order to approach these concepts from an empirical standpoint. Otherwise we will not be able to unlock new strata of knowledge. This includes the combination of different methods, the utilization of specific methods in a different context, as well as the development of novel methods.
What is however clear is that the three concepts - agency, complexity and emergence - have consequences about our premises of empirical knowledge. What if ultimately nothing is generalisable? What if all valid arguments are only valid for a certain time? And what if some strata will forever escape a truly reliable measurement? We cannot answer these problems here, yet it is important to differentiate what we know, what we may be able to know, and what we will probably never know. The uncertainty principle of Heisenberg in Quantum mechanics which refers to the the position and momentum of particles illustrates that some things cannot be approximated, observed or known. Equal claims can be made about larger phenomena, such as personal identity. Hence, as much as agency, complex systems and emergence can be boundary objects for methods, they equally highlight our (current) limitations.
The way forward
If we want to empirically investigate agency, we first and foremost investigate individuals, or actions of homogeneous entities, or consequences of actions of individuals. All this has consequences for the methods we apply, and the questions whether we observe or test premises has in addition further methodologial ramifications. I can interview individuals, yet this will hardly allow me to prove agency. Because of this, much of our current knowledge of agency is either rooted in widely deductive testing of very clear hypotheses, or questions of either logic or metaphysics, which are mostly associated with philosophy.
The investigation of complex systems has thrived in the last decades, both from an empirical as well as from a conceptual perspective. Many methods emerged or were subsequently adapted to answer questions as well as explore relations, and this thrive towards a deeper understanding of systems is at least one important difference to agency from a methodological standpoint. Much novel data is available, and often inductively explored. The scale of complex systems makes an intervention with a focus on causality a challenge, hence many investigated relations are purely correlative. Take for instance social media, or economic flows, which can be correlatively investigated, yet causality is an altogether different matter. This creates a methodological challenge concerning the fundamental assumptions that govern the interpretations of scientists. Many of our scientific questions are normative and many researchers assume causality in their investigations. This is at least partly because they are part of a discipline or tradition that assumes causality, and this makes them part of a normative group of scientists following this assumption. While this is trivial in itself, it illustrates that simply being part of a group of scientists that assumes causality cannot be a valid argument to actually confirm a causal relation. These two notions differ.
Another methodological problem related to causality are non-linear relations, since much of the statistical canon is based on probability and linear relations. While linear relations allow for a better inference of causal explanations, the long existing yet until recently hardly explored Bayesian statistics are an example that we can inductively learn about systems at a growing pace without being dependent on linearity or normal-distributions. This Bayesian revolution is currently under way, but much of the disciplines relying on statistics did not catch up on this yet. Other methodological approaches will certainly be explored to gain insight into the nuts and bolts of complex systems, yet this is only slowly emerging.
The whole globe - although not a closed system – can be seen as a global system, and this is certainly worthwhile pursuing from a methodological standpoint. Still, global dynamics consist of such diverse data, that simply the translational act to bring different data together seems almost impossible right now. While emergence can lead to novel solutions, globalisation and technology have triggered uncountable events of emergence, such as global conflicts, climate change, increase in cancer rates and biodiversity loss. Humankind did certainly not plan these potential endpoints of ourselves, instead they emerged out of unpredictable combinations of our actions. From a methodological standpoint, these events are just as unpredictable as is the effect which two molecules have onto each other and the environment. Emergence is a truly cross-scalar phenomenon. Consequently, many methodological accounts to counteract threats to human societies are correlative if they are empirical. We are far away from any deep understanding of emergence, and what makes phenomena emergent.
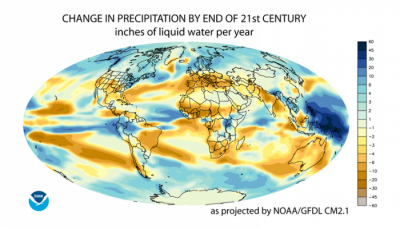
Based on the current methodological canon we can say: Much is not (yet?) observed, much is not known, and it will be crucial to understand what we will not be able to know, at least for the time being. These three different qualities of knowledge clearly highlight that research will have to collaborate - agency, many complex systems and several phenomena that are emergent cannot be sufficiently investigated by one discipline alone. While chemistry may find emergence in chemical reactions, and medicine may find interactions between different drugs, these results become normative as soon as they are applicable in the real world. To this claim, one could make the exception of ethics that are inherently normative. More broadly speaking, only philosophy can meaningfully engage with all three domains of knowledge - unobserved, not known and never known - yet may not be able to contribute to all empirical problems. This means, everything except philosophy may be able to go just into two of the three realms, the un-observed and unknown. We need to combine our methodological approaches to create the knowledge that is needed now. The need for collaboration is a question of responsibility, and only if all disciplines dissolve (or at least redefine their main goal to unite and not differentiate), agency may be fully explored and complex problems may be solved. Many of the solutions we have in our hands right now already would seem like wizardry to our ancestors. It is our responsibility to continue on this path, not as technocrats or positivists that have arrogant pride of their achievements, but as mere contributors to a wider debate that should ultimately encompass all society.
To say it with Derek Parfit:
"Some things (...) matter, and there are better and worse way to live. After many thousands of years of responding to reasons in ways that helped them to survive and reproduce, human beings can now respond to other reasons. We are a part of a Universe that is starting to understand itself. And we can partly understand, not only what is in fact true, but also what ought to be true, and what we might be able to make true. What now matters the most is that we avoid ending human history. If there are no rational beings elsewhere, it may depend on us and our successors whether it will all be worth it, because the existence of the Universe will have been on the whole good."
Further Information
- A video by Kurzgesagt that illustrates the concept of Emergence
- Calarco, Matthew. 2015. "Thinking through Animals - Identity, Difference, Indistinction" (An introduction to Critical Animal Studies to counter human-centered viewpoints)
- Parfit, Derek. 1984. "Reasons and Persons"
The author of this entry is Henrik von Wehrden.