Simple Statistical Tests
Method categorization | ||
---|---|---|
Quantitative | Qualitative | |
Inductive | Deductive | |
Individual | System | Global |
Past | Present | Future |
In short: Citizen Science is most commonly understood as a form of (environmental) data gathering that is done by amateur enthusiasts and analyzed by scientists.
Background
Simple testing statistics provide the baseline for advanced statistical thinking. While they are not so much used today within empirical analysis, simple tests are the foundation of modern statistics. The student t-test which originated around 100 years ago provided the crucial link from the more inductive thinking of Sir Francis Bacon towards the testing of hypotheses and the actual statistical testing of hypotheses. The formulation of the so-called null hypothesis is the first step within simple tests. Informed from theory this test calculates the probability whether the sample confirms the hypothesis or not. Null hypotheses are hence the assumptions we have about the world, and these assumptions can be confirmed or rejected.
What the method does
one sample t-test
It allows for the comparison of a sample to a pre-defined reference value. It tells you wether the mean of your sample differs significantly from this value. For this purpose, the t-test gives you a p-value at the end. If the p-value is below 0,05, the sample differs significantly from the reference value.
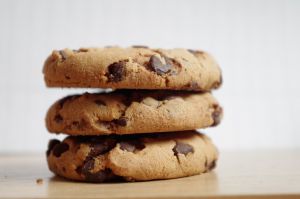
Example: Do the packages of your favourite cookie brand always contain as many cookies as stated on the outside of the box? Collect some of the packages, weigh the cookies contained therin and calculate the mean weight. You can compare this value now to the weight that is stated on the box using a one sample t-test.
two sample t-test
It allows a comparison of two different datasets or samples within an experiment. It tells you if the means of the two datasets differ significantly. If the p-value is below 0,05, the two samples differ significantly.
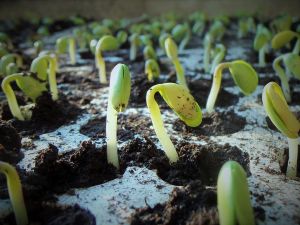
Example: The classic example would be to grow several plants and to add fertiliser to half of them. We can now compare the gross of the plants between the control samples without fertiliser and the samples that had fertiliser added. Plants with fertiliser (cm): 7.44 6.35 8.52 11.40 10.48 11.23 8.30 9.33 9.55 10.40 8.36 9.69 7.66 8.87 12.89 10.54 6.72 8.83 8.57 7.75 Plants without fertiliser (cm): 6.07 9.55 5.72 6.84 7.63 5.59 6.21 3.05 4.32 8.27 6.13 7.92 4.08 7.33 9.91 8.35 7.26 6.08 5.81 8.46 The result of the two-sample t-test is a p-value of 7.468e-05, which is close to zero and definetly below 0,05. Hence, the samples differ significantly and the fertilizer is likely to have an effect.
Paired t-test
It allows for a comparison of a sample before and after an intervention. Within such an experimental setup specific individuals are compared before and after an event. If the sample changes significantly, comparing start and end state, you will receive again a p-value below 0,05.
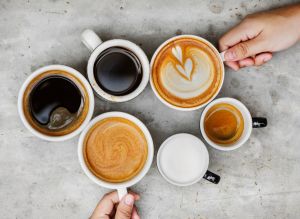
Example: concentration in the morning before or after a coffee.
Wilcoxon test
It is also a paired test (s. paired t-test), but you can use it if your sample is (exceptionally) NOT normally distributed, e.g. if one sample is skewed or if there are large gaps in the data. Regardless of these issues, the test will tell you if the means of two samples differ significantly (i.e. p-value below 0,05) by using ranks.
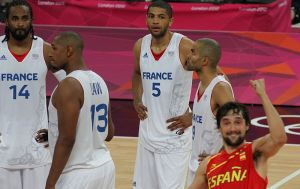
Example: Imagine you have a sample where half of your people are professional basketball players then the real size of people would not make sense. Therefore, as a robust measure in modern statistics rank tests were introduced.
Chi-square test of stochastic independence
If you observe an event and measure two variables, this test helps you to check if one variable influences the other one or if they occur independently from each other.
Example: Do the children of parents with an academic degree visit more often a university? The H0 hypothesis would be: The variables are independent from each other.
The H1 hypotheses would be: The variables influence each other. For example because children from better educated families have higher chances to achieve good results in school.
For this example, the chi-quare test yields a p-value of 2.439e-07, which is close to zero. We can reject the null hypothesis H0 and assume that, based on our sample, the education of parents has an influence on the education of their children.
f-test
The test allows you to compare the variances of two samples. If the p-value is lower than 0,05, the variances differ significantly.
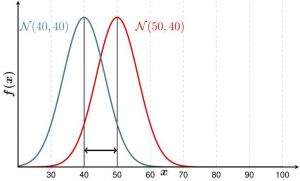
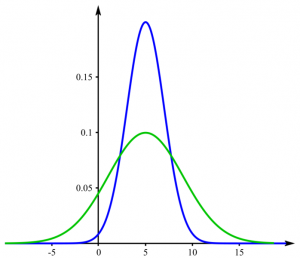
Example: If you examine players in a basketball and a hockey team, you would expect their heights to be different on average. But maybe the variance is not. Consider figure no. 1, where the mean is different, but the variance the same - this could be the case for your hockey and basketball team. In contrast, the height could be distributed as shown in figure no. 2. The f-test then would probably yield a p-value below 0,05.
Strengths & Challenges
- Citizen Science allows for access to data that would otherwise be inaccessible (covering long periods of time and wide spatial arrays) (6, 8).
- There is an educational quality to Citizen Science: participants not only learn about the respective subjects but also about scientific work (see Normativity).
- The research questions addressed through Citizen Science (in form of amateur data gathering) must take into consideration that most contributors will not be able to assess complex data. Instead, simple counting or observations can be expected (4). "Projects demanding high skill levels from participants can be successfully developed, but they require significant participant training and support materials such as training videos" (Bonney et al. 2009, p.979).
- In the case of bird watching (but also similar data types), errors may result from participants confusing bird species. This must be addressed through additional information material for the amateur scientists. (4)
- Citizen science always demands some sort of supra-infrastructure or project, since the data cannot be analysed by the participating citizens
Normativity
Complexity of the method and the analysed data
- The research questions addressed through Citizen Science (in form of amateur data gathering) must take into consideration that most contributors will not be able to assess complex data. Instead, simple counting or observations can be expected. "Projects demanding high skill levels from participants can be successfully developed, but they require significant participant training and support materials such as training videos" (Bonney et al. 2009, p.979).
Everything normative related to this method
- A strong bias may be imposed on the data due to divergent understandings of the data gathering procedure in the respective amateur individuals, diminishing the objectivity, reliability & validity of the process. This (alleged) lack of quality in the data gathered by amateurs has led to dispute over the validity of the method for scientific investigation and publication, which is to date an ongoing debate (6). A sample error can be minimized, for instance through additional information material, training and understandable, precise protocols for data collection (4, 6, 8).
- While researchers gain data through Citizen Science, the involved citizens get involved in scientific processes, strengthening their scientific literacy and learning about the subjects their are participating in (4, 7). This can also lead to positive social impacts, enabling communities to address local issues in a scientific manner and providing agency or even empowerment‚ to the respective actors (6).
- Citizen Science is a method of transdisciplinary research since it includes public actors into research processes. This element induces some normative notions that are addressed in the wiki entry on transdisciplinarity.
Outlook
Open questions for the method
- The diversity of terms and lack of precise definition should be overcome in the future to improve the application of Citizen Science (6, 7).
- The same is stated with regards to the redundancy in Citizen Science projects. Instead of designing new projects from the ground, functioning projects should be applied to new areas of interest (6).
Possible future developments - thoughts about the future of the method
- "Citizen Science (...) has over the past decade become a very influential and widely discussed concept with many scientists and commentators seeing it as the future of genuine interactive and inclusive science engagement (...)" (Riesch & Potter 2014, p.107). As new areas of academia develop participatory research designs, the potential of these approaches are becoming more and more visible (8). Therefore, after years of development and despite a lack of definite conceptualization, Citizen Science should be taken seriously and supported from more areas within academia (8).
- The spreading of Citizen Science approaches to local communities may support the incorporation of traditional knowledge into science policy and improve the science-society relationship in the future (6).
- The growing global availability of internet access improves amateurs' access to Citizen Science projects and increases the potential availability of data for researchers (7).
Key Publications
Theoretical
Irwin, A. 1995. Citizen Science: A Study of People, Expertise and Sustainable Development. London: Routledge.
- Debates the role of the public in science and vice versa, focusing on matters of risk and sustainable development.
Kullenberg, C. Kasperowski, D. 2016. What is Citizen Science? - A Scientometric Meta-Analysis. PLoS One 11(1)
- Examines the different conceptualizations and uses of Citizen Science in close to 2000 articles.
Bonney, R. et al. 2009. Citizen Science: A Developing Tool for Expanding Science knowledge and Scientific Literacy. BioScience 59(11).
- Describes an explanatory model for developing a citizen science project.
Empirical
Evans, C., E. Abrams, R. Reitsma, K. Roux, L. Salmonsen, and P. P. Marra. 2005. The neighborhood nestwatch program: participant outcomes of a citizen-science ecological research project. Conservation Biology 19:589–594
- Focuses on the scientific literacy outcomes of the citizens involved in a Citizen Science project.
References
(1) Kullenberg, C. Kasperowski, D. 2016. What is Citizen Science? - A Scientometric Meta-Analysis. PLoS One 11(1)
Further information
The author of this entry is Christopher Franz.