Difference between revisions of "Statistics and mixed methods"
(18 intermediate revisions by 4 users not shown) | |||
Line 1: | Line 1: | ||
− | + | '''Note:''' This entry revolves around mixed methods in statistics. For more on mixed methods in general, please refer to the entry on [[Mixed Methods]]. | |
− | |||
− | [[File:Bildschirmfoto 2020-06-14 um 17.59.31.png|thumb|This picture shows a brief history of statistics and its most important methods. For a more detailed look look at their [http://www.statslife.org.uk/images/pdf/timeline-of-statistics.pdf website]]] | + | ==Mixed methods== |
− | Mixed methods are one step in this evolution. [https://www.theguardian.com/science/2012/aug/19/thomas-kuhn-structure-scientific-revolutions Kuhn] spoke of scientific revolutions, which sounds appealing to many. As much as I like the underlying principle, I think that mixed methods are more of a scientific evolution that is slowly creeping in. The scientific canon that formed during the enlightenment and that was forged by the industrialisation and a cornerstone of modernity. The problems that arose out of this in modern science were slowly inching in between the two world wars, while methodology and especially statistics not only bloomed in full blossom, but [https://blog.udemy.com/importance-of-statistics/ contributed their part to the catastrophe]. Science opened up to new forms of knowledge, and while statistics would often contribute to such emerging arenas as psychology and clinical trials, other methodological approaches teamed up with statistics. [https://www.simplypsychology.org/interviews.html#structured Interviews] and surveys utilised statistics to unleash new sampling combined with statistics. Hence statistics teamed up with new developments, yet other approaches that were completely independent of statistics were also underway. Hence, new knowledge was unlocked, and science thrived into uncharted territory. | + | '''Much in modern science is framed around statistics, for better or worse.''' Due to the arrogance of "the scientific method" being labeled based on deductive approaches, and the fact that much of the early methodological approaches were biased to and dominated by quantitative approaches. This changed partly with the rise or better increase of qualitative methods during the last decades. We should realise to this end, that the development of the methodological canon is not independent but interconnected with the societal paradigm. Hence the abundance, development and diversity of the methodological canon is in a continuous [[Glossary|feedback loop]] with changes in society, but also driven from changes in society. Take the rise of experimental designs, and the growth it triggered through fostering developments in agriculture and medicine, for better or worse. Another example are the severe developments triggered by [https://www.thoughtco.com/critical-theory-3026623 critical theory], which had clearly ramifications towards the methodological canon, and the societal developments during this time. Despite all these ivory towers is science not independent from the Zeitgeist and the changes within society, and science is often both rooted and informed from the past and influence the presence, while also building futures. This is the great privilege society gained from science, that we can now create, embed and interact with a new knowledge production that is ever evolving.<br/> |
+ | |||
+ | [[File:Bildschirmfoto 2020-06-14 um 17.59.31.png|1000px|center|thumb|'''This picture shows a brief history of statistics and its most important methods.''' For a more detailed look look at their [http://www.statslife.org.uk/images/pdf/timeline-of-statistics.pdf website]]]<br/> | ||
+ | |||
+ | '''Mixed methods are one step in this evolution.''' [https://www.theguardian.com/science/2012/aug/19/thomas-kuhn-structure-scientific-revolutions Kuhn] spoke of scientific revolutions, which sounds appealing to many. As much as I like the underlying principle, I think that mixed methods are more of a scientific evolution that is slowly creeping in. The scientific canon that formed during the enlightenment and that was forged by the industrialisation and a cornerstone of modernity. The problems that arose out of this in modern science were slowly inching in between the two world wars, while methodology and especially statistics not only bloomed in full blossom, but [https://blog.udemy.com/importance-of-statistics/ contributed their part to the catastrophe]. Science opened up to new forms of knowledge, and while statistics would often contribute to such emerging arenas as psychology and clinical trials, other methodological approaches teamed up with statistics. [https://www.simplypsychology.org/interviews.html#structured Interviews] and surveys utilised statistics to unleash new sampling combined with statistics. Hence statistics teamed up with new developments, yet other approaches that were completely independent of statistics were also underway. Hence, new knowledge was unlocked, and science thrived into uncharted territory. | ||
From a systematic standpoint we can now determine at least three developments: 1) Methods that were genuinely new, 2) methods that were used in a novel context, and 3) methods that were combined with other methods. Let us embed statistics into this line of thinking. Much of the general line of thinking in statistics in the last phase of modernity, i.e. before the two world wars. While in terms of more advanced statistics of course much was developed later and is still being developed, but some of the large breakthroughs in terms of the general line of thinking were rather early. In other words, in terms of what is most abundantly being applied up until the computer age, much was already developed early in the 20th century. Methods in statistics were still developed after that, but were often so complicated that they only increased in application once computers became widely available. | From a systematic standpoint we can now determine at least three developments: 1) Methods that were genuinely new, 2) methods that were used in a novel context, and 3) methods that were combined with other methods. Let us embed statistics into this line of thinking. Much of the general line of thinking in statistics in the last phase of modernity, i.e. before the two world wars. While in terms of more advanced statistics of course much was developed later and is still being developed, but some of the large breakthroughs in terms of the general line of thinking were rather early. In other words, in terms of what is most abundantly being applied up until the computer age, much was already developed early in the 20th century. Methods in statistics were still developed after that, but were often so complicated that they only increased in application once computers became widely available. | ||
− | What was however quite relevant for statistics was the emergence into diverse disciplines. Many scientific fields implemented statistics into their development to a point that it was dominating much of the discourse (ecology, psychology, economics), and often this also led to specific applications of statistics and even genuinely new approaches down the road. | + | What was however quite relevant for statistics was the [[Glossary|emergence]] into diverse disciplines. Many scientific fields implemented statistics into their development to a point that it was dominating much of the discourse (ecology, psychology, economics), and often this also led to specific applications of statistics and even genuinely new approaches down the road. |
Where statistics also firmly extended their map on the landscape of methods was in the combination with other methods. Structured Interviews and surveys are a standard example where many approaches served for the gathering of data and the actual analysis is conducted by statistics. Hence new revolutionary methods often directly implemented statistics into their utilisation, making the footing of statistics even more firm. | Where statistics also firmly extended their map on the landscape of methods was in the combination with other methods. Structured Interviews and surveys are a standard example where many approaches served for the gathering of data and the actual analysis is conducted by statistics. Hence new revolutionary methods often directly implemented statistics into their utilisation, making the footing of statistics even more firm. | ||
+ | |||
+ | |||
+ | For more on the development of new methods, please refer to the entries on [[History of Methods]] as well as [[Questioning the status quo in methods]]. | ||
==Triangulation of statistics within methodological ontologies== | ==Triangulation of statistics within methodological ontologies== | ||
[[File:Multiple-perspectives.gif|thumb|right|This wonderful picture from [https://proswrite.com/2013/02/26/what-is-plain-language-part-four-putting-it-all-together-in-a-process/ prowrite.com] shows how different methods can help to illuminate different aspects of a phenomenon. Triangulation is about bringing the different perspectives together, use them in harmony.]] | [[File:Multiple-perspectives.gif|thumb|right|This wonderful picture from [https://proswrite.com/2013/02/26/what-is-plain-language-part-four-putting-it-all-together-in-a-process/ prowrite.com] shows how different methods can help to illuminate different aspects of a phenomenon. Triangulation is about bringing the different perspectives together, use them in harmony.]] | ||
− | [https://ebn.bmj.com/content/22/3/67 Triangulation] is often interpreted in a purely quantitative sense, which is not true. I would use it here in a sense of combination of different methods to triangulate knowledge from diverse sources. Ideally, triangulation allows to create more knowledge than with single method approaches. More importantly, [http://www.qualres.org/HomeTria-3692.html triangulation] should allow to use methods in harmony, meaning the sum of the methods that are triangulated is more than the sum of its parts. To this end, triangulation can be applied in many contexts, and may bring more precision to situations where mixed methods are not enough to describe the methodological design to enable for a clear communication of the research design or approach. | + | [https://ebn.bmj.com/content/22/3/67 Triangulation] is often interpreted in a purely quantitative sense, which is not true. I would use it here in a sense of combination of different methods to triangulate knowledge from diverse sources. '''Ideally, triangulation allows to create more knowledge than with single method approaches.''' More importantly, [http://www.qualres.org/HomeTria-3692.html triangulation] should allow to use methods in harmony, meaning the sum of the methods that are triangulated is more than the sum of its parts. To this end, triangulation can be applied in many contexts, and may bring more precision to situations where mixed methods are not enough to describe the methodological design to enable for a clear communication of the research design or approach. |
Not least because of the dominating position that statistics held in modern science, and also because of the lack of other forms of knowledge becoming more and more apparent, qualitative methods were increasingly developed and utilised after the second world war. I keep this dating so vague, not because of my firm belief that much was a rather continuous evolvement, but mostly because of the end of modernity. With the enlightenment divinely ending, we entered a new age where science became more open, and methods became more interchangeable within different branches of science. | Not least because of the dominating position that statistics held in modern science, and also because of the lack of other forms of knowledge becoming more and more apparent, qualitative methods were increasingly developed and utilised after the second world war. I keep this dating so vague, not because of my firm belief that much was a rather continuous evolvement, but mostly because of the end of modernity. With the enlightenment divinely ending, we entered a new age where science became more open, and methods became more interchangeable within different branches of science. | ||
− | ==Quantitative vs qualitative== | + | == Mixed Methods and Design Criteria == |
+ | '''Note:''' For more details on the categories below, please refer to the entry on [[Design Criteria of Methods]]. | ||
+ | |||
+ | ====Quantitative vs qualitative==== | ||
[[File:Bildschirmfoto 2020-06-14 um 18.03.39.png|thumb|This picture emphasizes the main differences between quantitative and qualitative research. But do we really need to either decide for the one or the another?]] | [[File:Bildschirmfoto 2020-06-14 um 18.03.39.png|thumb|This picture emphasizes the main differences between quantitative and qualitative research. But do we really need to either decide for the one or the another?]] | ||
− | |||
− | ==Inductive vs deductive== | + | The opening of a world of quantitative knowledge started a discourse between [https://www.simplypsychology.org/qualitative-quantitative.html quantitative and qualitative research] that has effectively never ended. Pride, self esteem and ignorance are words that come to my mind if I try to characterise what I observe still today in the exchange between these two lines of thought. Only when we establish [[Glossary|trust]] and appreciation, we can ultimately bring these two complementary types of knowledge together. From the standpoint of someone educated in statistics I can only say that it is my hope that words such as [https://sustainabilitymethods.org/index.php/Misunderstood_concepts_in_statistics "significant", "correlated", "clustered"] are not used unthoughtfully, but as the analysis approaches and associated concepts that these words stand for. What is even more difficult to me is when these concepts are rejected altogether as outdated, dogmatic, or plain wrong. Only if we can join forces between these two schools of thought, we may solve the challenges we face. |
+ | Likewise, it is not sound that many people educated in statistics plainly reject qualitative knowledge, and are dogmatic in their slumber. '''I consider the gap between these two worlds as one of the biggest obstacles for development in science.''' People educated in statistics should be patient in both explaining their approaches and results, and being receptive and open minded about other forms of knowledge. There is still a lot of work in order to bridge this gap. If we cannot bridge it, we need to walk around the gap. Connect through concrete action, and create joined knowledge, which may evolve into a joined learning. Explain statistics to others, but in a modest, and open approach. | ||
+ | |||
+ | ====Inductive vs deductive==== | ||
[[File:Bildschirmfoto 2020-06-14 um 18.07.47.png|thumb|The Leuphana and also many other research institutes conduct a board of ethics before a study starts.]] | [[File:Bildschirmfoto 2020-06-14 um 18.07.47.png|thumb|The Leuphana and also many other research institutes conduct a board of ethics before a study starts.]] | ||
− | A fairly similar situation can be diagnosed for the difference between [https://conjointly.com/kb/deduction-and-induction/ inductive and deductive approaches]. Many people building on theory are on a high horse, and equally claim many inductive researchers to be the only ones to approach science in the most correct manner. I think both sides are right and wrong at the same time. What can be clearly said for everybody versatile in statistics is, that both sides are lying. The age of big data crashed with many disciplines that were once theory driven. While limping behind the modern era, these disciplines are often pretending to build hypotheses, merely because their community and scientific journals demand a line of thinking that is build on hypotheses testing. Since these disciplines have long had access to large datasets that are analysed in an inductive fashion, people pretend to write hypothesis, when they formulated them in fact after the whole analysis was finalised. While this may sound horrific to many, scientists were not more than frogs in the boiling pot, slowly getting warmer. This system slowly changed or adapted, until no one really realised that we were on the wrong track. There are antidotes, such as [https://www.cos.io/our-services/prereg preregistration] of studies in medicine and psychology, yet we are far way from solving this problem. | + | |
+ | A fairly similar situation can be diagnosed for the difference between [https://conjointly.com/kb/deduction-and-induction/ inductive and deductive approaches]. Many people building on theory are on a high horse, and equally claim many inductive researchers to be the only ones to approach science in the most correct manner. '''I think both sides are right and wrong at the same time.''' What can be clearly said for everybody versatile in statistics is, that both sides are lying. The age of big data crashed with many disciplines that were once theory driven. While limping behind the modern era, these disciplines are often pretending to build hypotheses, merely because their community and scientific journals demand a line of thinking that is build on hypotheses testing. Since these disciplines have long had access to large datasets that are analysed in an inductive fashion, people pretend to write [[Glossary|hypothesis]], when they formulated them in fact after the whole analysis was finalised. While this may sound horrific to many, scientists were not more than frogs in the boiling pot, slowly getting warmer. This system slowly changed or adapted, until no one really realised that we were on the wrong track. There are antidotes, such as [https://www.cos.io/our-services/prereg preregistration] of studies in medicine and psychology, yet we are far way from solving this problem. | ||
+ | |||
Equally, many would argue that researchers claim to be inductive in their approach, when they are in fact not only biased all over the place, but also widely informed by previous study and theory. Many would claim, that this is more a weak point of qualitative methods, but I would disagree. With the wealth of data that became available over the last decades though the internet, we also have in statistics much to our disposal, and many claim to be completely open minded about their analysis, when in fact the suffer also from many types of biases, and are equally stuck in a dogmatic slumber when they claim to be free and unbiased. | Equally, many would argue that researchers claim to be inductive in their approach, when they are in fact not only biased all over the place, but also widely informed by previous study and theory. Many would claim, that this is more a weak point of qualitative methods, but I would disagree. With the wealth of data that became available over the last decades though the internet, we also have in statistics much to our disposal, and many claim to be completely open minded about their analysis, when in fact the suffer also from many types of biases, and are equally stuck in a dogmatic slumber when they claim to be free and unbiased. | ||
Line 28: | Line 41: | ||
This is why it is so important to write an outline about your research, preregister studies if possible, and have an ethical check being conducted if necessary. We compiled the [https://www.leuphana.de/forschung/transparenz-in-der-forschung/gute-wissenschaftliche-praxis.html application form for the board of ethics from Leuphana University] at the end of this Wiki. | This is why it is so important to write an outline about your research, preregister studies if possible, and have an ethical check being conducted if necessary. We compiled the [https://www.leuphana.de/forschung/transparenz-in-der-forschung/gute-wissenschaftliche-praxis.html application form for the board of ethics from Leuphana University] at the end of this Wiki. | ||
− | ==Spatial and temporal scales== | + | ====Spatial and temporal scales==== |
[[File:Bildschirmfoto 2020-06-14 um 18.12.31.png|thumb|Network analysis can be applied if you want to know how all your actors are tied together which relationships occur between themen and who has how much influence.]] | [[File:Bildschirmfoto 2020-06-14 um 18.12.31.png|thumb|Network analysis can be applied if you want to know how all your actors are tied together which relationships occur between themen and who has how much influence.]] | ||
Statistics can be utilised basically across all spatial and temporal scales. Global economic dynamics are correlated, experiments are conducted on plant individuals, and surveys are conducted within systems. This showcases why statistics got established across all spatial scales, but instantly also highlights once more that statistics can only offer a part of the picture. More complex statistical analysis such as [https://www.statisticssolutions.com/structural-equation-modeling/ structural equation models] and [https://www.jessesadler.com/post/network-analysis-with-r/ network analysis] are currently emerging, allowing for a more holistic system perspective as part of a statistical analysis. The rise of big data allows us to make connections between different data sources, and hence bridge different forms of knowledge, but also different spatial scales. While much of these statistical tools were established decades ago, we only slowly start to compile datasets that allow for such analysis. | Statistics can be utilised basically across all spatial and temporal scales. Global economic dynamics are correlated, experiments are conducted on plant individuals, and surveys are conducted within systems. This showcases why statistics got established across all spatial scales, but instantly also highlights once more that statistics can only offer a part of the picture. More complex statistical analysis such as [https://www.statisticssolutions.com/structural-equation-modeling/ structural equation models] and [https://www.jessesadler.com/post/network-analysis-with-r/ network analysis] are currently emerging, allowing for a more holistic system perspective as part of a statistical analysis. The rise of big data allows us to make connections between different data sources, and hence bridge different forms of knowledge, but also different spatial scales. While much of these statistical tools were established decades ago, we only slowly start to compile datasets that allow for such analysis. | ||
Likewise, with the increasing availability of more and more data, an increasing diversity of temporal dimensions are emerging, and statistics such as panel statistics and [https://ourcodingclub.github.io/tutorials/mixed-models/#what mixed effect models] allow for an evolving understanding of change like never before. Past data is equally being explored in order to predict the future. We need to be aware that statistics offers only a predictive picture here, and is unable to implement future changes that are not implemented into the analysis. We predict the future to the best of our knowledge, but we will only know the future, once it becomes our presence, which is trivial in general, but makes predictions notoriously difficult. | Likewise, with the increasing availability of more and more data, an increasing diversity of temporal dimensions are emerging, and statistics such as panel statistics and [https://ourcodingclub.github.io/tutorials/mixed-models/#what mixed effect models] allow for an evolving understanding of change like never before. Past data is equally being explored in order to predict the future. We need to be aware that statistics offers only a predictive picture here, and is unable to implement future changes that are not implemented into the analysis. We predict the future to the best of our knowledge, but we will only know the future, once it becomes our presence, which is trivial in general, but makes predictions notoriously difficult. | ||
− | To this end, statistics needs to be aware of its own limitations, and needs to be critical of the knowledge it produces in order to contribute valid knowledge. Statistical results have a certain confidence, results can be significant, even parsimonious, yet may only offer a part of the picture. [https://sustainabilitymethods.org/index.php/ | + | |
+ | To this end, statistics needs to be aware of its own limitations, and needs to be critical of the knowledge it produces in order to contribute valid knowledge. '''Statistical results have a certain confidence, results can be significant, even parsimonious, yet may only offer a part of the picture.''' [https://sustainabilitymethods.org/index.php/Non-equilibrium_dynamics#The_world_can_.28not.29_be_predicted Unexplained variance] may be an important piece of the puzzle in a mixed method approach, as it helps us to understand how much we do not understand. Statistics may be taken more serious if it clearly highlights the limitations it has or reveals. We need to be careful to not only reveal the patterns we may understand through statistics, but also how these patterns are limited in terms of a holistic understanding. However, there is also reason for optimism when it comes to statistics. Some decades ago, statistics were exclusive to a small fraction of scientists that could afford a very expensive computer, or calculations were made by hand. Today, more and more people have access to computers, and as a consequence to powerful software that allows for statistical analysis. The rise of computers led to an incredible amount of statistical knowledge being produced, and the internet enabled the spread of this knowledge across the globe. We may be living in a world of exponential growths, and while many dangers are connected to this recognition, the rise in knowledge cannot be a bad, at least not all of it. More statistical knowledge bears hope for less ignorance, but this demands as well the responsibility of [[Glossary|communicating]] results clearly and precisely, also highlighting gaps in and limitations of our knowledge. | ||
==Possibilities of interlinkages== | ==Possibilities of interlinkages== | ||
[[File:Bildschirmfoto 2020-06-14 um 18.16.39.png|thumb|Scenario planning is a popular method in interdiscplinary and transdisciplinary research. It combines systemic knowledge about the current state and normative target knowledge of the desired future state.]] | [[File:Bildschirmfoto 2020-06-14 um 18.16.39.png|thumb|Scenario planning is a popular method in interdiscplinary and transdisciplinary research. It combines systemic knowledge about the current state and normative target knowledge of the desired future state.]] | ||
− | We only start to understand how we can combine statistical methods with other forms of knowledge that are used in parralel. Sequential combination of other methods with statistics has been long known, as the example of interviews and their statistical analysis has already shown. However, the parallel integration of knowledge gained through statistics and other methods is still in its infancy. [https://www.forbes.com/sites/stratfor/2015/01/08/scenario-planning-and-strategic-forecasting/#766d057e411a Scenario planing] is a prominent example that can integrate diverse forms of knowledge; other approaches are slowly investigated. However, the integrational capability needed by a team to combine such different forms of knowledge is still rare, and research or better scientific publications are only slowly starting to combine diverse methods in parralel. Hence many papers that claim a mixed method approach often actually mean either a sequential approach or different methods that are unconnected. While this is perfectly valid and a step forward, science will have to go a long way to combine parralel methods and their knowledge more deeply. Funding schemes in science are still widely disciplinary, and this dogma often dictates a canon of methods that may have proven its value, some may argue. However these approaches did not substantially create the normative knowledge that is needed to contribute towards a sustainable future. | + | We only start to understand how we can combine statistical methods with other forms of knowledge that are used in parralel. Sequential combination of other methods with statistics has been long known, as the example of interviews and their statistical analysis has already shown. '''However, the parallel integration of knowledge gained through statistics and other methods is still in its infancy.''' [https://www.forbes.com/sites/stratfor/2015/01/08/scenario-planning-and-strategic-forecasting/#766d057e411a Scenario planing] is a prominent example that can integrate diverse forms of knowledge; other approaches are slowly investigated. However, the integrational capability needed by a team to combine such different forms of knowledge is still rare, and research or better scientific publications are only slowly starting to combine diverse methods in parralel. Hence many papers that claim a mixed method approach often actually mean either a sequential approach or different methods that are unconnected. While this is perfectly valid and a step forward, science will have to go a long way to combine parralel methods and their knowledge more deeply. [[Glossary|Funding]] schemes in science are still widely disciplinary, and this dogma often dictates a canon of methods that may have proven its value, some may argue. However these approaches did not substantially create the normative knowledge that is needed to contribute towards a sustainable future. |
− | + | ==Bokeh== | |
[[File:Bildschirmfoto 2020-06-14 um 19.24.14.png|thumb|The picture allows us very clearly to depict a person. We can see a lot of details about this person, such as the clothes, a not too cheap umbrella, and much more. We can slo see that it is obviously in a part of the world where is rains, where you have public transportation, both tram and buses. We have high buildings, but also low building, traffic, street lights, hence a lot of information that can be depicted despite the blurriness.]] | [[File:Bildschirmfoto 2020-06-14 um 19.24.14.png|thumb|The picture allows us very clearly to depict a person. We can see a lot of details about this person, such as the clothes, a not too cheap umbrella, and much more. We can slo see that it is obviously in a part of the world where is rains, where you have public transportation, both tram and buses. We have high buildings, but also low building, traffic, street lights, hence a lot of information that can be depicted despite the blurriness.]] | ||
One of the nicest metaphors of interlinking methods is the japanese word Bokeh. Bokeh means basically depth of field, which is the effect when you have a really beautiful camera lens, and keep the aperture - the opening of the lens - very wide. Photographs made in this setting typically have a laser sharp foreground, and a beautifully blurry background. You have one distance level very crisp, and the other like a washed watercolour matrix. A pro photographer or tec geek will appreciate such a photo with a "Whoa, nice Bokeh". Mixed method designs can be set up in a similar way. While you have one method focussing on something in the foreground, other methods can give you a blurry understanding of the background. Negotiating and designing this role in a mixed method setting is central in order to clarify which method demands which depth and focus, to follow with the allegory of photography. Way too often we demand each method having the same importance in a mixed method design. More often than not I am not sure if this is actually possible, or even desirable. Instead, I would suggest to stick to the Bokeh design, and harmonise what is in the foreground, and what is in the background. Statistics may give you some general information about a case study setting and its background, but deep open interviews may allow for the focus and depth needed to really understand the dynamics in a case study. | One of the nicest metaphors of interlinking methods is the japanese word Bokeh. Bokeh means basically depth of field, which is the effect when you have a really beautiful camera lens, and keep the aperture - the opening of the lens - very wide. Photographs made in this setting typically have a laser sharp foreground, and a beautifully blurry background. You have one distance level very crisp, and the other like a washed watercolour matrix. A pro photographer or tec geek will appreciate such a photo with a "Whoa, nice Bokeh". Mixed method designs can be set up in a similar way. While you have one method focussing on something in the foreground, other methods can give you a blurry understanding of the background. Negotiating and designing this role in a mixed method setting is central in order to clarify which method demands which depth and focus, to follow with the allegory of photography. Way too often we demand each method having the same importance in a mixed method design. More often than not I am not sure if this is actually possible, or even desirable. Instead, I would suggest to stick to the Bokeh design, and harmonise what is in the foreground, and what is in the background. Statistics may give you some general information about a case study setting and its background, but deep open interviews may allow for the focus and depth needed to really understand the dynamics in a case study. | ||
− | An example of a paper where a lot of information was analysed that is interconnected is [https://www.ecologyandsociety.org/vol19/iss4/art32/ Hanspach et al 2014], which contains scenarios, causal loop analysis, GIS, and much more methodological approaches that are interlinked. | + | An example of a paper where a lot of information was analysed that is interconnected is [https://www.ecologyandsociety.org/vol19/iss4/art32/ Hanspach et al 2014], which contains scenarios, [[System Thinking & Causal Loop Diagrams|causal-loop analysis]], [[Geographical Information Systems|GIS]], and much more methodological approaches that are interlinked. |
− | ==Statistics and disciplines== | + | |
+ | ==Statistics and disciplines== | ||
[[File:Bildschirmfoto 2020-06-14 um 19.34.30.png|thumb|Disciplines in science differentate themselves in many many subcategories. Thinking in disciplines can be seen as double-edged sword. They create specific knowledge but on the other hand not looking behind your own horizon is problematic.]] | [[File:Bildschirmfoto 2020-06-14 um 19.34.30.png|thumb|Disciplines in science differentate themselves in many many subcategories. Thinking in disciplines can be seen as double-edged sword. They create specific knowledge but on the other hand not looking behind your own horizon is problematic.]] | ||
Statistics are deeply embedded in the DNA of many scientific disciplines, and strangely enough is the rejection of statistics as a valid method by many disciplines that associate themselves with critical thinking. | Statistics are deeply embedded in the DNA of many scientific disciplines, and strangely enough is the rejection of statistics as a valid method by many disciplines that associate themselves with critical thinking. | ||
− | I think statistics should be utilised when they provide a promising outcome. Due to the formation and [https://www.youtube.com/watch?v=YvtCLceNf30&list=PL8dPuuaLjXtNppY8ZHMPDH5TKK2UpU8Ng&t=0s development of scientific disciplines], this is however hardly the case. Instead, we have many disciplines that evolved around and based on statistics, such as psychology, ecology, (empirical) social science or parts of economics. All these scientific disciplines sooner or later encountered struggles with statistics. Examples are models when better statistics becoming more and more complicated, hence violating parsimony which led to the crisis questioning the value of probability driven statistics. Many disciplines evolved from deductive schools of thought, but with more and more data becoming available, this closed into a mishmash of inductive and deductive thinking, which I have mentioned before. Yet another problem is embedded into causality, as many studies from economics can not rely on causal links, yet inform policy and politics as if they are investigating nothing but causal links. | + | '''I think statistics should be utilised when they provide a promising outcome.''' Due to the formation and [https://www.youtube.com/watch?v=YvtCLceNf30&list=PL8dPuuaLjXtNppY8ZHMPDH5TKK2UpU8Ng&t=0s development of scientific disciplines], this is however hardly the case. Instead, we have many disciplines that evolved around and based on statistics, such as psychology, ecology, (empirical) social science or parts of economics. All these scientific disciplines sooner or later encountered struggles with statistics. Examples are models when better statistics becoming more and more complicated, hence violating parsimony which led to the crisis questioning the value of probability driven statistics. Many disciplines evolved from deductive schools of thought, but with more and more data becoming available, this closed into a mishmash of inductive and deductive thinking, which I have mentioned before. Yet another problem is embedded into [https://sustainabilitymethods.org/index.php/Causality causality], as many studies from economics can not rely on causal links, yet inform policy and politics as if they are investigating nothing but causal links. |
Many scientific disciplines question the value of statistics, which is important. However, while statistics only offer a piece of the puzzle, rejecting statistics altogether is equally harsh. Instead, we ought to add precision in communicating the limitations of statistical results and evolve the utilisation of approaches to build trust between disciplines into our agenda and learning. A more fundamental question is most deeply embedded in philosophy, if I were to name a discipline. Philosophy still deals with the most fundamental questions such as "Is there an objective way to act?" or "Are there normative facts?". These questions illustrate why statistics will ultimately need to team up more deeply with philosophy to solve these questions. [https://lux.leuphana.de/vufind/Record/1696677149 "Making comparisons count"] is an example for a work from philosophy that clearly informs statistics, and more importantly, the results that are reported from statistical analysis. | Many scientific disciplines question the value of statistics, which is important. However, while statistics only offer a piece of the puzzle, rejecting statistics altogether is equally harsh. Instead, we ought to add precision in communicating the limitations of statistical results and evolve the utilisation of approaches to build trust between disciplines into our agenda and learning. A more fundamental question is most deeply embedded in philosophy, if I were to name a discipline. Philosophy still deals with the most fundamental questions such as "Is there an objective way to act?" or "Are there normative facts?". These questions illustrate why statistics will ultimately need to team up more deeply with philosophy to solve these questions. [https://lux.leuphana.de/vufind/Record/1696677149 "Making comparisons count"] is an example for a work from philosophy that clearly informs statistics, and more importantly, the results that are reported from statistical analysis. | ||
− | Methods are at the heart of the disciplinary dogma. I postulate that the method that started them all -philosophy- will be able to solve this problem, but | + | '''Methods are at the heart of the disciplinary dogma.''' I postulate that the method that started them all - philosophy - will be able to solve this problem, but it is not clear when this will happen. Sustainability science is an example of a solution oriented and diverse arena that tries to approach solutions to this end, yet this is why many scientists that define themselves through their discipline reject it. [[Glossary|Dialogue]] will be necessary, and we need to understand that deep focus is possible even for diverse approaches. Learning a method truly takes about as much time as learning a musical instrument, hence thousands of hours of hours at least. Yet while some researchers will be pivotal in integrating diverse approaches to research, the majority will probably commit to a deeper focus. However, appreciation and respect for other forms of knowledge should become part of the academic curriculum, or should be on the agenda even earlier. |
To cut a very long story short, scientific disciplines arose out of a need for a specific labour force, the need for specific types of knowledge, and the surplus in resources that came out of the colonies, among many other reasons. I hope that we may overcome our differences and the problems that arise out of disciplinary identities, and focus on knowledge and solutions instead. Time will tell how we explore this possibility. | To cut a very long story short, scientific disciplines arose out of a need for a specific labour force, the need for specific types of knowledge, and the surplus in resources that came out of the colonies, among many other reasons. I hope that we may overcome our differences and the problems that arise out of disciplinary identities, and focus on knowledge and solutions instead. Time will tell how we explore this possibility. | ||
− | =External Links= | + | ==External Links== |
− | ==Articles== | + | ====Articles==== |
[https://www.thoughtco.com/critical-theory-3026623 Critical Theory]: an example of mixed methods | [https://www.thoughtco.com/critical-theory-3026623 Critical Theory]: an example of mixed methods | ||
Line 91: | Line 106: | ||
[https://www.forbes.com/sites/stratfor/2015/01/08/scenario-planning-and-strategic-forecasting/#766d057e411a Scenario Planning]: The most important steps | [https://www.forbes.com/sites/stratfor/2015/01/08/scenario-planning-and-strategic-forecasting/#766d057e411a Scenario Planning]: The most important steps | ||
− | ==Videos== | + | ====Videos==== |
[https://www.youtube.com/watch?v=YvtCLceNf30&list=PL8dPuuaLjXtNppY8ZHMPDH5TKK2UpU8Ng&t=0s The History of Science]: A very interesting YouTube series | [https://www.youtube.com/watch?v=YvtCLceNf30&list=PL8dPuuaLjXtNppY8ZHMPDH5TKK2UpU8Ng&t=0s The History of Science]: A very interesting YouTube series | ||
+ | ---- | ||
+ | [[Category:Statistics]] | ||
+ | |||
+ | The [[Table of Contributors|author]] of this entry is Henrik von Wehrden. |
Latest revision as of 07:25, 20 September 2021
Note: This entry revolves around mixed methods in statistics. For more on mixed methods in general, please refer to the entry on Mixed Methods.
Contents
Mixed methods
Much in modern science is framed around statistics, for better or worse. Due to the arrogance of "the scientific method" being labeled based on deductive approaches, and the fact that much of the early methodological approaches were biased to and dominated by quantitative approaches. This changed partly with the rise or better increase of qualitative methods during the last decades. We should realise to this end, that the development of the methodological canon is not independent but interconnected with the societal paradigm. Hence the abundance, development and diversity of the methodological canon is in a continuous feedback loop with changes in society, but also driven from changes in society. Take the rise of experimental designs, and the growth it triggered through fostering developments in agriculture and medicine, for better or worse. Another example are the severe developments triggered by critical theory, which had clearly ramifications towards the methodological canon, and the societal developments during this time. Despite all these ivory towers is science not independent from the Zeitgeist and the changes within society, and science is often both rooted and informed from the past and influence the presence, while also building futures. This is the great privilege society gained from science, that we can now create, embed and interact with a new knowledge production that is ever evolving.
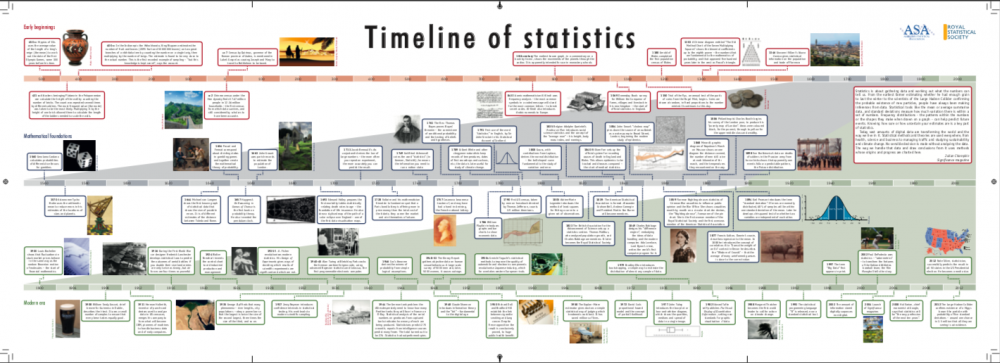
Mixed methods are one step in this evolution. Kuhn spoke of scientific revolutions, which sounds appealing to many. As much as I like the underlying principle, I think that mixed methods are more of a scientific evolution that is slowly creeping in. The scientific canon that formed during the enlightenment and that was forged by the industrialisation and a cornerstone of modernity. The problems that arose out of this in modern science were slowly inching in between the two world wars, while methodology and especially statistics not only bloomed in full blossom, but contributed their part to the catastrophe. Science opened up to new forms of knowledge, and while statistics would often contribute to such emerging arenas as psychology and clinical trials, other methodological approaches teamed up with statistics. Interviews and surveys utilised statistics to unleash new sampling combined with statistics. Hence statistics teamed up with new developments, yet other approaches that were completely independent of statistics were also underway. Hence, new knowledge was unlocked, and science thrived into uncharted territory.
From a systematic standpoint we can now determine at least three developments: 1) Methods that were genuinely new, 2) methods that were used in a novel context, and 3) methods that were combined with other methods. Let us embed statistics into this line of thinking. Much of the general line of thinking in statistics in the last phase of modernity, i.e. before the two world wars. While in terms of more advanced statistics of course much was developed later and is still being developed, but some of the large breakthroughs in terms of the general line of thinking were rather early. In other words, in terms of what is most abundantly being applied up until the computer age, much was already developed early in the 20th century. Methods in statistics were still developed after that, but were often so complicated that they only increased in application once computers became widely available.
What was however quite relevant for statistics was the emergence into diverse disciplines. Many scientific fields implemented statistics into their development to a point that it was dominating much of the discourse (ecology, psychology, economics), and often this also led to specific applications of statistics and even genuinely new approaches down the road. Where statistics also firmly extended their map on the landscape of methods was in the combination with other methods. Structured Interviews and surveys are a standard example where many approaches served for the gathering of data and the actual analysis is conducted by statistics. Hence new revolutionary methods often directly implemented statistics into their utilisation, making the footing of statistics even more firm.
For more on the development of new methods, please refer to the entries on History of Methods as well as Questioning the status quo in methods.
Triangulation of statistics within methodological ontologies
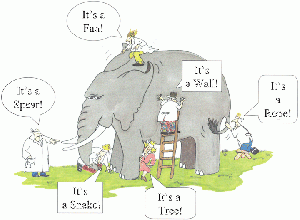
Triangulation is often interpreted in a purely quantitative sense, which is not true. I would use it here in a sense of combination of different methods to triangulate knowledge from diverse sources. Ideally, triangulation allows to create more knowledge than with single method approaches. More importantly, triangulation should allow to use methods in harmony, meaning the sum of the methods that are triangulated is more than the sum of its parts. To this end, triangulation can be applied in many contexts, and may bring more precision to situations where mixed methods are not enough to describe the methodological design to enable for a clear communication of the research design or approach.
Not least because of the dominating position that statistics held in modern science, and also because of the lack of other forms of knowledge becoming more and more apparent, qualitative methods were increasingly developed and utilised after the second world war. I keep this dating so vague, not because of my firm belief that much was a rather continuous evolvement, but mostly because of the end of modernity. With the enlightenment divinely ending, we entered a new age where science became more open, and methods became more interchangeable within different branches of science.
Mixed Methods and Design Criteria
Note: For more details on the categories below, please refer to the entry on Design Criteria of Methods.
Quantitative vs qualitative
The opening of a world of quantitative knowledge started a discourse between quantitative and qualitative research that has effectively never ended. Pride, self esteem and ignorance are words that come to my mind if I try to characterise what I observe still today in the exchange between these two lines of thought. Only when we establish trust and appreciation, we can ultimately bring these two complementary types of knowledge together. From the standpoint of someone educated in statistics I can only say that it is my hope that words such as "significant", "correlated", "clustered" are not used unthoughtfully, but as the analysis approaches and associated concepts that these words stand for. What is even more difficult to me is when these concepts are rejected altogether as outdated, dogmatic, or plain wrong. Only if we can join forces between these two schools of thought, we may solve the challenges we face. Likewise, it is not sound that many people educated in statistics plainly reject qualitative knowledge, and are dogmatic in their slumber. I consider the gap between these two worlds as one of the biggest obstacles for development in science. People educated in statistics should be patient in both explaining their approaches and results, and being receptive and open minded about other forms of knowledge. There is still a lot of work in order to bridge this gap. If we cannot bridge it, we need to walk around the gap. Connect through concrete action, and create joined knowledge, which may evolve into a joined learning. Explain statistics to others, but in a modest, and open approach.
Inductive vs deductive
A fairly similar situation can be diagnosed for the difference between inductive and deductive approaches. Many people building on theory are on a high horse, and equally claim many inductive researchers to be the only ones to approach science in the most correct manner. I think both sides are right and wrong at the same time. What can be clearly said for everybody versatile in statistics is, that both sides are lying. The age of big data crashed with many disciplines that were once theory driven. While limping behind the modern era, these disciplines are often pretending to build hypotheses, merely because their community and scientific journals demand a line of thinking that is build on hypotheses testing. Since these disciplines have long had access to large datasets that are analysed in an inductive fashion, people pretend to write hypothesis, when they formulated them in fact after the whole analysis was finalised. While this may sound horrific to many, scientists were not more than frogs in the boiling pot, slowly getting warmer. This system slowly changed or adapted, until no one really realised that we were on the wrong track. There are antidotes, such as preregistration of studies in medicine and psychology, yet we are far way from solving this problem.
Equally, many would argue that researchers claim to be inductive in their approach, when they are in fact not only biased all over the place, but also widely informed by previous study and theory. Many would claim, that this is more a weak point of qualitative methods, but I would disagree. With the wealth of data that became available over the last decades though the internet, we also have in statistics much to our disposal, and many claim to be completely open minded about their analysis, when in fact the suffer also from many types of biases, and are equally stuck in a dogmatic slumber when they claim to be free and unbiased.
To this end, statistics may rise to a level where a clearer documentation and transparency enables a higher level of science, that is also aware of the fact that knowledge changes. This is a normal process in science, and does not automatically make previous results wrong. Instead, these results, even if they are changing, are part of the picture. This is why it is so important to write an outline about your research, preregister studies if possible, and have an ethical check being conducted if necessary. We compiled the application form for the board of ethics from Leuphana University at the end of this Wiki.
Spatial and temporal scales
Statistics can be utilised basically across all spatial and temporal scales. Global economic dynamics are correlated, experiments are conducted on plant individuals, and surveys are conducted within systems. This showcases why statistics got established across all spatial scales, but instantly also highlights once more that statistics can only offer a part of the picture. More complex statistical analysis such as structural equation models and network analysis are currently emerging, allowing for a more holistic system perspective as part of a statistical analysis. The rise of big data allows us to make connections between different data sources, and hence bridge different forms of knowledge, but also different spatial scales. While much of these statistical tools were established decades ago, we only slowly start to compile datasets that allow for such analysis.
Likewise, with the increasing availability of more and more data, an increasing diversity of temporal dimensions are emerging, and statistics such as panel statistics and mixed effect models allow for an evolving understanding of change like never before. Past data is equally being explored in order to predict the future. We need to be aware that statistics offers only a predictive picture here, and is unable to implement future changes that are not implemented into the analysis. We predict the future to the best of our knowledge, but we will only know the future, once it becomes our presence, which is trivial in general, but makes predictions notoriously difficult.
To this end, statistics needs to be aware of its own limitations, and needs to be critical of the knowledge it produces in order to contribute valid knowledge. Statistical results have a certain confidence, results can be significant, even parsimonious, yet may only offer a part of the picture. Unexplained variance may be an important piece of the puzzle in a mixed method approach, as it helps us to understand how much we do not understand. Statistics may be taken more serious if it clearly highlights the limitations it has or reveals. We need to be careful to not only reveal the patterns we may understand through statistics, but also how these patterns are limited in terms of a holistic understanding. However, there is also reason for optimism when it comes to statistics. Some decades ago, statistics were exclusive to a small fraction of scientists that could afford a very expensive computer, or calculations were made by hand. Today, more and more people have access to computers, and as a consequence to powerful software that allows for statistical analysis. The rise of computers led to an incredible amount of statistical knowledge being produced, and the internet enabled the spread of this knowledge across the globe. We may be living in a world of exponential growths, and while many dangers are connected to this recognition, the rise in knowledge cannot be a bad, at least not all of it. More statistical knowledge bears hope for less ignorance, but this demands as well the responsibility of communicating results clearly and precisely, also highlighting gaps in and limitations of our knowledge.
Possibilities of interlinkages
We only start to understand how we can combine statistical methods with other forms of knowledge that are used in parralel. Sequential combination of other methods with statistics has been long known, as the example of interviews and their statistical analysis has already shown. However, the parallel integration of knowledge gained through statistics and other methods is still in its infancy. Scenario planing is a prominent example that can integrate diverse forms of knowledge; other approaches are slowly investigated. However, the integrational capability needed by a team to combine such different forms of knowledge is still rare, and research or better scientific publications are only slowly starting to combine diverse methods in parralel. Hence many papers that claim a mixed method approach often actually mean either a sequential approach or different methods that are unconnected. While this is perfectly valid and a step forward, science will have to go a long way to combine parralel methods and their knowledge more deeply. Funding schemes in science are still widely disciplinary, and this dogma often dictates a canon of methods that may have proven its value, some may argue. However these approaches did not substantially create the normative knowledge that is needed to contribute towards a sustainable future.
Bokeh
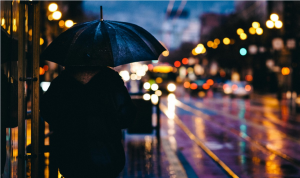
One of the nicest metaphors of interlinking methods is the japanese word Bokeh. Bokeh means basically depth of field, which is the effect when you have a really beautiful camera lens, and keep the aperture - the opening of the lens - very wide. Photographs made in this setting typically have a laser sharp foreground, and a beautifully blurry background. You have one distance level very crisp, and the other like a washed watercolour matrix. A pro photographer or tec geek will appreciate such a photo with a "Whoa, nice Bokeh". Mixed method designs can be set up in a similar way. While you have one method focussing on something in the foreground, other methods can give you a blurry understanding of the background. Negotiating and designing this role in a mixed method setting is central in order to clarify which method demands which depth and focus, to follow with the allegory of photography. Way too often we demand each method having the same importance in a mixed method design. More often than not I am not sure if this is actually possible, or even desirable. Instead, I would suggest to stick to the Bokeh design, and harmonise what is in the foreground, and what is in the background. Statistics may give you some general information about a case study setting and its background, but deep open interviews may allow for the focus and depth needed to really understand the dynamics in a case study.
An example of a paper where a lot of information was analysed that is interconnected is Hanspach et al 2014, which contains scenarios, causal-loop analysis, GIS, and much more methodological approaches that are interlinked.
Statistics and disciplines
Statistics are deeply embedded in the DNA of many scientific disciplines, and strangely enough is the rejection of statistics as a valid method by many disciplines that associate themselves with critical thinking.
I think statistics should be utilised when they provide a promising outcome. Due to the formation and development of scientific disciplines, this is however hardly the case. Instead, we have many disciplines that evolved around and based on statistics, such as psychology, ecology, (empirical) social science or parts of economics. All these scientific disciplines sooner or later encountered struggles with statistics. Examples are models when better statistics becoming more and more complicated, hence violating parsimony which led to the crisis questioning the value of probability driven statistics. Many disciplines evolved from deductive schools of thought, but with more and more data becoming available, this closed into a mishmash of inductive and deductive thinking, which I have mentioned before. Yet another problem is embedded into causality, as many studies from economics can not rely on causal links, yet inform policy and politics as if they are investigating nothing but causal links.
Many scientific disciplines question the value of statistics, which is important. However, while statistics only offer a piece of the puzzle, rejecting statistics altogether is equally harsh. Instead, we ought to add precision in communicating the limitations of statistical results and evolve the utilisation of approaches to build trust between disciplines into our agenda and learning. A more fundamental question is most deeply embedded in philosophy, if I were to name a discipline. Philosophy still deals with the most fundamental questions such as "Is there an objective way to act?" or "Are there normative facts?". These questions illustrate why statistics will ultimately need to team up more deeply with philosophy to solve these questions. "Making comparisons count" is an example for a work from philosophy that clearly informs statistics, and more importantly, the results that are reported from statistical analysis.
Methods are at the heart of the disciplinary dogma. I postulate that the method that started them all - philosophy - will be able to solve this problem, but it is not clear when this will happen. Sustainability science is an example of a solution oriented and diverse arena that tries to approach solutions to this end, yet this is why many scientists that define themselves through their discipline reject it. Dialogue will be necessary, and we need to understand that deep focus is possible even for diverse approaches. Learning a method truly takes about as much time as learning a musical instrument, hence thousands of hours of hours at least. Yet while some researchers will be pivotal in integrating diverse approaches to research, the majority will probably commit to a deeper focus. However, appreciation and respect for other forms of knowledge should become part of the academic curriculum, or should be on the agenda even earlier.
To cut a very long story short, scientific disciplines arose out of a need for a specific labour force, the need for specific types of knowledge, and the surplus in resources that came out of the colonies, among many other reasons. I hope that we may overcome our differences and the problems that arise out of disciplinary identities, and focus on knowledge and solutions instead. Time will tell how we explore this possibility.
External Links
Articles
Critical Theory: an example of mixed methods
Thomas Kuhn: The way he changed science
The Importance of Statistics: A look into different disciplines
Interviews: The way statistics influenced interviews
Triangulation: A detailed article with lots of examples
Triangulation II: An overview
Qualitative and Quantitative Research: A comparison
Deduction and Induction: The differences
Short explanation and the possibility for further reading: Preregistration
What they do and application forms: The Leuphana Board of Ethics
Guidelines for good scientific practive: Leuphana University
Structural Equation Modeling: An introduction
Network Analysis: An inroduction with an analysis in R
Linear Mixed Models: An introduction with R code
Scenario Planning: The most important steps
Videos
The History of Science: A very interesting YouTube series
The author of this entry is Henrik von Wehrden.